
Human monoclonal antibodies against Shigella (ShiMabs), for therapy and vaccine acceleration
The team's activity in the project consists of developing AI-based methods for evaluating monoclonal antibodies' activities against pathogens and improving the monoclonal antibodies design.
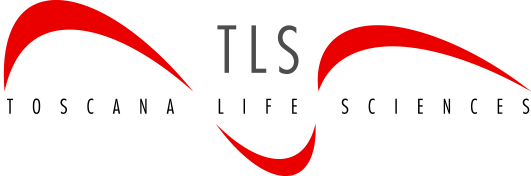
OVERVIEW
One of the most pressing challenges in modern medicine is antimicrobial resistance (AMR), which poses a significant threat to public health worldwide. As traditional antibiotics become less effective against resistant strains of bacteria, innovative therapeutic approaches are critical.
Monoclonal antibodies (mAbs) offer a promising solution by providing targeted action against specific pathogens, potentially circumventing the mechanisms of resistance that plague conventional antimicrobial agents. By harnessing the specificity and potency of mAbs, we can develop new treatments that enhance the body’s immune response and reduce reliance on broad-spectrum antibiotics. To identify potential therapeutic mAbs, a high number must be tested and screened, which can be labor-intensive and time-consuming with current methods.
GOALS
The project aims to develop deep learning techniques for analysing biological images and videos. The study of the interaction of bacteria with the host is an integral part of vaccine design and is usually performed using cell models and ad-hoc microscopy, often with non-scalable approaches that drastically reduce the throughput of the methodology and its application for strong antimicrobial resistance. To evaluate antibodies' effectiveness against bacterial strains, we are developing methods combining image and video analysis and machine learning/deep learning techniques. The project’s goals fulfil the national need for protocols and intelligent tools for discovering therapy resistance mechanisms and new molecules against infectious agents for developing monoclonal antibodies and vaccines.
Project Info
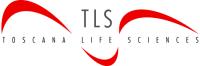