
LEarning the Geometry of knOwledge in AI systems
LEGO.AI aims at the exploitation of Geometric Deep Learning (GDL) for the purpose of combining the recent advancements in the subfields of Continual Learning (CL), Domain Adaptation (DA), Few Shot Learning (FSL) and Lifelong Learning (LLL).
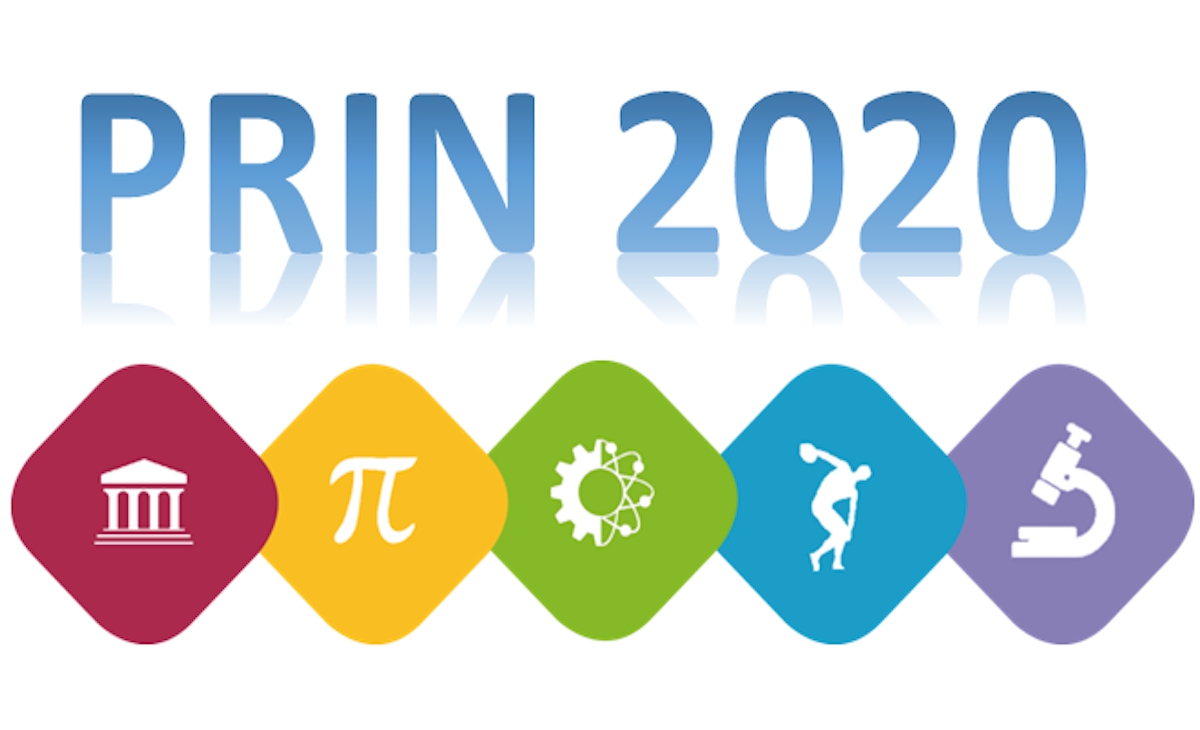
LEGO.AI (LEarning the Geometry of knOwledge in AI systems) is linked to the recently approved Progetto di Ricerca di Interesse Nazionale (PRIN). The project aims to develop a new generation of AI systems, able to learn from data without unrealistic assumptions regarding data and annotation availability. Indeed, it is becoming increasingly evident the need for systems that possess human-like capabilities of learning incrementally, generalizing from a few examples, and efficiently adapting to unknown environments. The goal of the project is thus to lay the foundations of a novel theory, embracing the concepts of GDL to provide lifelong learning capabilities to machines and consequently build a new set of algorithms able to deal with the aforementioned setting.
Current state of the art family of models are limited in a number of important aspects. Most notably, they lack the possibility of properly exploiting the hidden structure of the data and often treat data as ordinary vectors with no geometric meaning. We propose instead a substantial paradigm shift, placing back the geometric nature of the data into focus. Indeed, the applications of GDL for computer vision tasks and other sciences have been historically limited, thus a wide range of important problems dealing with online data, changing tasks, generalization, and data scarcity are still out of reach.
A central aspect that hinders the applicability of Deep Learning (DL) in real-world scenarios is the necessity for a large set of annotated data. New systems that aim for a broad industry use need to ensure that the few available observations are used efficiently, making sure that the efforts made to provide such data are rewarded. The project aims to investigate the application of geometric regularizers in the aforementioned scenarios to promote the training effectiveness of DL models. In particular, we propose the adoption of geometric priors to increase the generalization power of FSL models, which has not been investigated to date.
The key goal of the project is to develop a new family of models that would allow the exploitation of the geometric properties of the underlying data structure. Differently from existing techniques, forcing a geometric similarity between the latent spaces learned by CL, DA, and FSL methods introduce a new biologically plausible dimension to the traditional learning scheme, allowing the new family of models to cope with challenging domains spacing from health, security, and robotics. The output of this work will be i) a novel geometry-driven theoretical framework, and ii) an open-source software library compatible with current DL frameworks.
Partners: Università degli Studi di Parma, Università degli Studi di Catania, Università degli Studi di Modena e Reggio Emilia, Università degli Studi di Trento
Publications
1 | Boschini, Matteo; Bonicelli, Lorenzo; Porrello, Angelo; Bellitto, Giovanni; Pennisi, Matteo; Palazzo, Simone; Spampinato, Concetto; Calderara, Simone "Transfer without Forgetting" Proceedings of the 17th European Conference on Computer Vision, Tel Aviv, Israel, 23-27 Oct, 2022, 2022 | DOI: 10.1007/978-3-031-20050-2_40 Conference |
Project Info
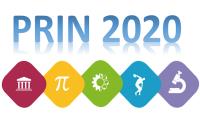