
AI for Earth Observation AIDEO
AIDEO is being developed with the purpose of developing an innovative, scalable and accurate process to produce West Nile Disease (WND) risk maps, using Earth Observation (EO) data and specific AI algorithms. Vector-borne diseases (VBDs) are an important threat with an increasing impact on public health due to wider geographic range of occurrence and higher incidences. West Nile virus is one of the most spread zoonotic VBD in Italy and Europe. Its transmission cycle is well understood, with birds acting as the primary hosts and mosquito vectors transmitting the virus to other birds, while humans and horses are occasional dead-end hosts. Identifying suitable environmental conditions across large areas containing multiple species of potential hosts and vectors can be difficult.
AI and EO are used as innovative methods for monitoring West Nile Virus spread. The recent and massive availability of EO data and the continuous development of innovative AI methods can be a great help to automatically identify patterns in big datasets and to make highly accurate predictions.

The synergy among historical surveillance data on West Nile Disease occurrences, highly detailed remotely sensed imagery and newly innovative artificial intelligence algorithms might be the perfect combination to explore hidden patterns and develop automated processes to predict WND outbreaks and derive risk maps. Data of WND cases derived by several epidemics that have been affecting the Italian territory since 2008.
Information regarding EO data to be used, criteria to select ground truth data, temporal interval to be analysed and different Deep Neural Network models are evaluated and defined. Selection criteria and preparation of remotely sensed products are then investigated, considering data from multiple sources, various sensors, spectral bands, spatial resolutions and revisit times. WND and EO data are selected to guarantee a correct spatial and temporal representation of the last ten-year epidemics. WND cases are extracted from the official repository of the Italian Ministry of Health (National Information System of Animal Disease Notification - SIMAN), validated and selected, in space and time, according to the requirements defined in the previous phase.
WND ground truth outbreaks are split in different datasets to be used to train and test the DNN model, then fine-tune the model and hence make predictions and evaluate the overall accuracy. Selected EO data are collected from different sources and stored in a centralised system that organises and pre-process them according to the requirements. Classical statistical models for WND spread (suitability analysis, logistic regression, etc.) are developed to be compared with AI model performance.
Press release from ESA:
http://down2earth.esa.int/2020/11/earth-observation-and-artificial-intelligence-for-global-health/
Publications
1 |
Vincenzi, Stefano; Porrello, Angelo; Buzzega, Pietro; Cipriano, Marco; Fronte, Pietro; Cuccu, Roberto; Ippoliti, Carla; Conte, Annamaria; Calderara, Simone
"The color out of space: learning self-supervised representations for Earth Observation imagery"
Proceedings of the 25th International Conference on Pattern Recognition,
Milan, Italy,
pp. 3034
-3041
,
10-15 January 2021,
2021
| DOI: 10.1109/ICPR48806.2021.9413112
Conference
![]() |
2 |
Candeloro, L.; Ippoliti, C.; Iapaolo, F.; Monaco, F.; Morelli, D.; Cuccu, R.; Fronte, P.; Calderara, S.; Vincenzi, S.; Porrello, A.; D'Alterio, N.; Calistri, P.; Conte, A.
"Predicting WNV circulation in Italy using earth observation data and extreme gradient boosting model"
REMOTE SENSING,
vol. 12,
pp. 3064
-3083
,
2020
| DOI: 10.3390/RS12183064
Journal
![]() |
Project Info
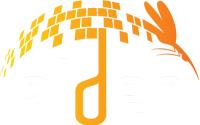