
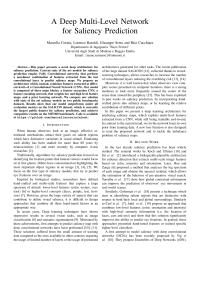
A Deep Multi-Level Network for Saliency Prediction
Abstract: This paper presents a novel deep architecture for saliency prediction. Current state of the art models for saliency prediction employ Fully Convolutional networks that perform a non-linear combination of features extracted from the last convolutional layer to predict saliency maps. We propose an architecture which, instead, combines features extracted at different levels of a Convolutional Neural Network (CNN). Our model is composed of three main blocks: a feature extraction CNN, a feature encoding network, that weights low and high level feature maps, and a prior learning network. We compare our solution with state of the art saliency models on two public benchmarks datasets. Results show that our model outperforms under all evaluation metrics on the SALICON dataset, which is currently the largest public dataset for saliency prediction, and achieves competitive results on the MIT300 benchmark.
Citation:
Cornia, Marcella; Baraldi, Lorenzo; Serra, Giuseppe; Cucchiara, Rita "A Deep Multi-Level Network for Saliency Prediction" Pattern Recognition (ICPR), 2016 23rd International Conference on, Cancun, Mexico, pp. 3488 -3493 , 4-8 Dec 2016, 2016 DOI: 10.1109/ICPR.2016.7900174
not available
Paper download:
- Author version:
- DOI: 10.1109/ICPR.2016.7900174