
Deep Renal Biopsy Immunofluorescence Image Analysis
Immunohistopathology is an essential technique in the diagnostic workflow of a kidney biopsy, we wanted to evaluate the role of a Convolutional Neural Network (CNN) as a support tool for kidney immunofluorescence reporting. High magnification (400X) immunofluorescence images of kidney biopsies performed from the year 2001 to 2018 were collected. The report, adopted at the Division of Nephrology of the AOU Policlinico di Modena, describes the specimen in terms of 'Appearance', 'Distribution', 'Location' and 'Intensity' of the glomerular deposits identified with fluorescent antibodies against IgG, IgA, IgM immunoglobulins, C1q and C3 complement fractions, Fibrinogen, kappa and lambda light chains. The report was used as ground truth for the training of the CNNs. 12259 immunofluorescence images of 2542 subjects undergoing kidney biopsy were collected, the test set analysis showed accuracy values between 0.79 ('Irregular Capillary wall' feature) and 0.94 ('Fine Granular' feature). The agreement test of the results obtained by the Convolutional Neural Networks with respect to the ground truth showed similar values to three pathologists of our center. Convolutional Neural Networks were 117.3 times faster than human evaluators in analyzing 180 image tests. A web platform, where it is possible to upload digitized images of immunofluorescence specimens, is available to evaluate the potential of our approach.
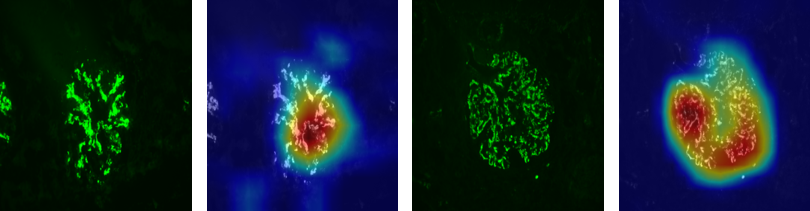
We trained the neural networks to recognize the four different ‘Locations’: mesangial, continuous regular capillary wall, discontinuous regular capillary wall, irregular capillary wall. A general class named capillary wall obtained by merging all the different kinds of capillary wall locations was added to the analysis. Regarding ‘Location’, the pattern recognition tasks are completely independent classification problems, since each image could present neither, none, or every combination of the investigated location characteristics. For each one of the tasks, a specific Convolutional Neural Network is trained to classify a single image as either presenting or not presenting the pattern of interest. The same approach is taken to tackle the deposit ‘Appearance’ (linear/pseudolinear, coarse granular and fine granular) and the deposit ‘Distribution’ (segmental and global). The main finding of our work is that Convolutional Neural Networks achieve an accurate definition of the main pathology features usually collected from this type of specimen: ‘Appearance’, ‘Distribution’, ‘Location’ and ‘Intensity’ of the deposits. The comparison of agreement among three pathologists shows a moderate agreement and, in this respect, Convolutional Neural Networks show a similar performance to human evaluators. The interest in Deep Learning applied to nephropathology has grown significantly in recent years. The researchers adopted Deep Learning technology to achieve different goals: some focused on the automated identification of the main microscopic structures (glomeruli, tubules, vessels etc.), some authors worked on the segmentation of the identified structures , and others have used Convolutional Neural Networks to obtain automated clinical classifications. We can consider our approach as part of the strategies for developing a tool for assisting in automated clinical classification. In our work we did not deal with the problem of the preliminary extraction of glomerular structures, that was already addressed in many previous papers. Since our dataset was already made up of high-magnification images of individual glomeruli historically collected in the last 18 years of manual reporting of the kidney specimens, we focused our attention on the pure classification of these images. Immunohistopathologic analysis (in particular the immunofluorescence approach) has never been previously addressed with Deep Learning techniques. We therefore focused on the ability to extract from these images the features normally collected by our pathologists during the examination. The immunofluorescence interpretation, like many other clinical imaging techniques, is a field in which the Deep Learning approach can offer a significant contribution. Indeed, any medical imaging technique involving human reporting is conditioned by a significant interpretative subjectivity. This poor reproducibility has been repeatedly documented in the old kidney histopathological literature and has been recently confirmed in studies concerning the application of Deep Learning in kidney pathology. The indices of agreement between human pathologists vary according to the metrics used, the preparation of the specimen, the histological parameters assessed, and the kidney disease considered; overall, reported agreement rate between human kidney pathologists is fair to moderate with agreement ratio ranging between 0.3 and 0.6. In this scenario, the Convolutional Neural Network algorithms have ground truth agreement performances similar to the human operators. Our data suggest a high accuracy in identifying the analyzed features, which varies from 0.79 to 0.94.
Publications
1 |
Pollastri, Federico; Maroņas, Juan; Bolelli, Federico; Ligabue, Giulia; Paredes, Roberto; Magistroni, Riccardo; Grana, Costantino
"Confidence Calibration for Deep Renal Biopsy Immunofluorescence Image Classification"
2020 25th International Conference on Pattern Recognition (ICPR),
Milan, Italy,
pp. 1298
-1305
,
Jan 10-15,
2021
| DOI: 10.1109/ICPR48806.2021.9412685
Conference
![]() |
2 |
Ligabue, Giulia; Pollastri, Federico; Fontana, Francesco; Leonelli, Marco; Furci, Luciana; Giovanella, Silvia; Alfano, Gaetano; Cappelli, Gianni; Testa, Francesca; Bolelli, Federico; Grana, Costantino; Magistroni, Riccardo
"Evaluation of the Classification Accuracy of the Kidney Biopsy Direct Immunofluorescence through Convolutional Neural Networks"
CLINICAL JOURNAL OF THE AMERICAN SOCIETY OF NEPHROLOGY,
vol. 15,
pp. 1445
-1454
,
2020
| DOI: 10.2215/CJN.03210320
Journal
![]() |