
Visualization Techniques for Explainable AI
Explaining neural network predictions can increase the transparency of models and help to justify correct and incorrect outputs in a human-friendly way. While there have been diverse attempts to provide explanations about the inference process in different forms, the Class Activation Mapping (CAM) approach has proven to be particularly effective in providing visual explanations by taking a weighted combination of activation maps from a convolutional layer. We focused on the enhancement of the evaluation and reproducibility of such approaches. To this aim, we designed a novel evaluation protocol to quantify explanation maps, which show better effectiveness and simplifies comparisons between approaches. Our proposed score, named ADCC, overcomes the limitation of existing metrics and jointly considers the correctness, coherency, and complexity of the explanation maps in a single-valued metric. Experiments have been conducted on five different CAM-based visualization methods and six backbones and have testified the appropriateness of the proposal its generality across different settings.
Paper
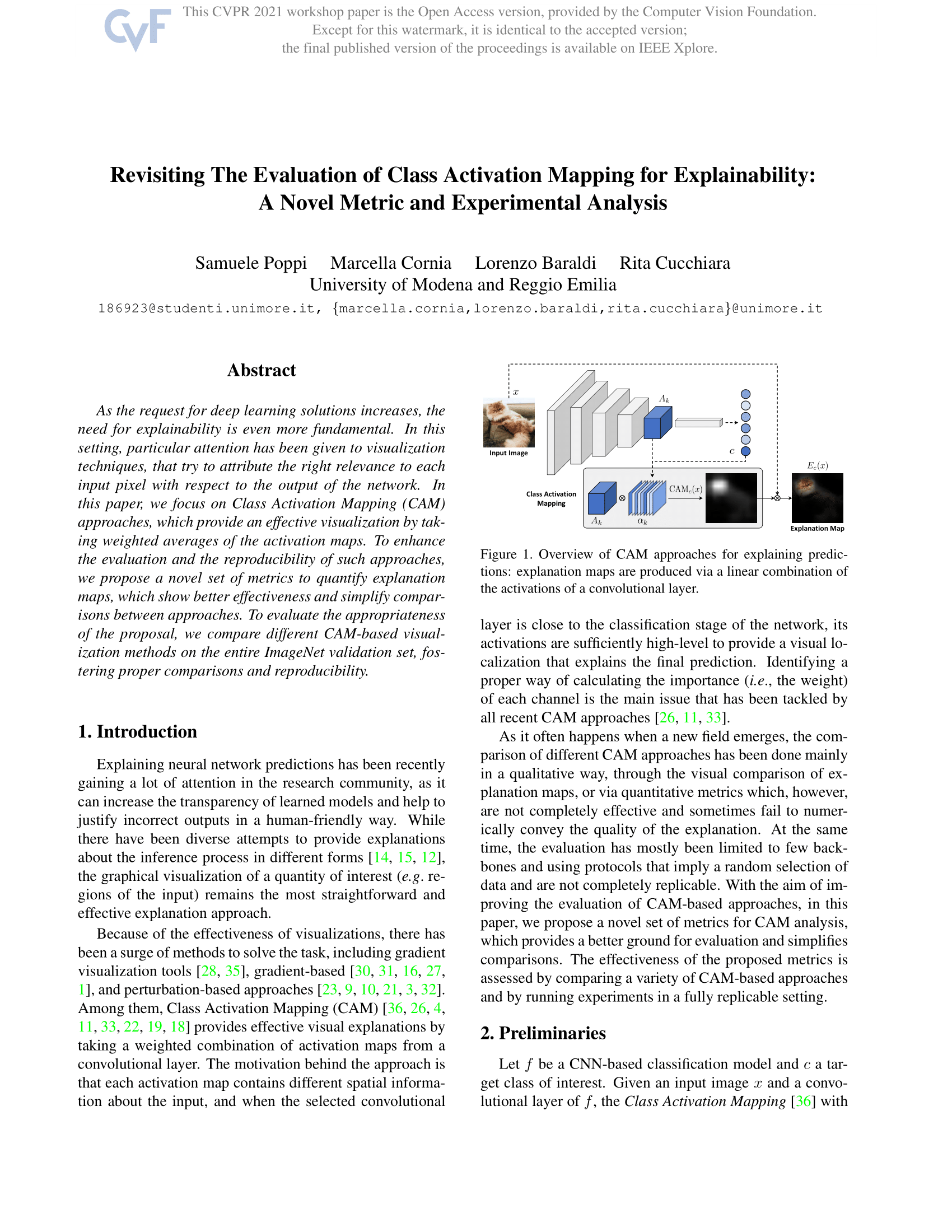
Revisiting The Evaluation of Class Activation Mapping for Explainability: A Novel Metric and Experimental Analysis
S.Poppi, M.Cornia, L.Baraldi, R.Cucchiara
CVPR'2021 Responsible Computer Vision Workshop
Bibtex
If you find our work useful for your research, please cite:
@inproceedings{poppi2021revisiting, title={Revisiting The Evaluation of Class Activation Mapping for Explainability: A Novel Metric and Experimental Analysis}, author={Poppi, Samuele and Cornia, Marcella and Baraldi, Lorenzo and Cucchiara, Rita}, booktitle={Proceedings of the IEEE/CVF Conference on Computer Vision and Pattern Recognition Workshops}, year={2021} }
Publications
1 |
Poppi, Samuele; Cornia, Marcella; Baraldi, Lorenzo; Cucchiara, Rita
"Revisiting The Evaluation of Class Activation Mapping for Explainability: A Novel Metric and Experimental Analysis"
2021 IEEE Computer Society Conference on Computer Vision and Pattern Recognition Workshops,
Virtual,
pp. 2299
-2304
,
June 19-25, 2021,
2021
| DOI: 10.1109/CVPRW53098.2021.00260
Conference
![]() |