
Skin Lesion Analysis
Skin lesion analysis has been one of the research fields of our labs for several years.
In particular, we made use of Convolutional Neural Networks (CNNs) to perform semantic segmentation and lesion classification on dermoscopic images. Moreover, we explored the usage of Generative Adversarial Networks (GANs) in the data augmentation process, and developed a tool for content-based dermoscopic image retrieval.
Beside writing numerous published papers we participated to the International Skin Imaging Collaboration (ISIC) challenge, achieving the third best result in 2019 in collaboration with the Universidad Politecnica de Valencia (UPV).
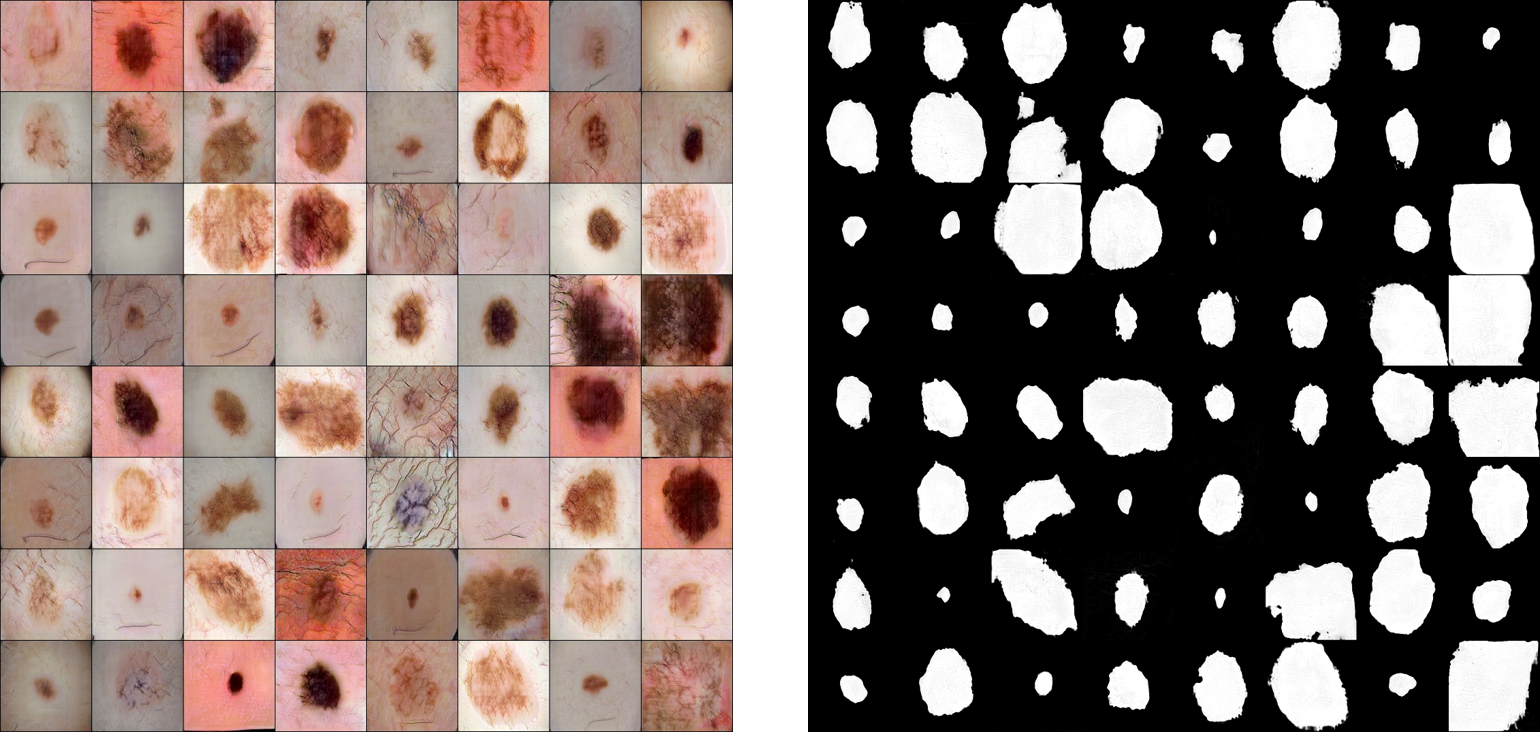
The segmentation task could greatly benefit from a bigger and wider dataset, hence we approached the problem focusing on the data augmentation process.
GANs are often used to create unlabeled examples, which cannot be directly employed for the training of a supervised algorithm. We improved the role of the GAN in the training process by implementing an architecture which generates both the image and its segmentation mask, making it extremely easy to exploit new synthetic images as additional training data. In the proposed GANs, both the input and the output are 4 channel images: the first three channels are the R, G and B components and the fourth one is the binary segmentation mask. This approach provides a tool for generating new skin lesion images coupled with a coherently learned segmentation mask.
Generative adversarial networks were proved to be able to produce high quality syinthetic images and, most importantly, segmentation masks that look very coherent with the respective generated images. As a matter of fact, pre-training CNNs with generated images effectively increased the segmentation accuracy.
Publications
1 |
Allegretti, Stefano; Bolelli, Federico; Pollastri, Federico; Longhitano, Sabrina; Pellacani, Giovanni; Grana, Costantino
"Supporting Skin Lesion Diagnosis with Content-Based Image Retrieval"
2020 25th International Conference on Pattern Recognition (ICPR),
Milan, Italy,
pp. 8053
-8060
,
Jan 10-15,
2021
| DOI: 10.1109/ICPR48806.2021.9412419
Conference
![]() |
2 |
Pollastri, Federico; Parreņo, Mario; Maroņas, Juan; Bolelli, Federico; Paredes, Roberto; Ramos, Daniel; Grana, Costantino
"A Deep Analysis on High Resolution Dermoscopic Image Classification"
IET COMPUTER VISION,
vol. 15,
pp. 514
-526
,
2021
| DOI: 10.1049/cvi2.12048
Journal
![]() |
3 |
Pollastri, Federico; Bolelli, Federico; Paredes Palacios, Roberto; Grana, Costantino
"Augmenting data with GANs to segment melanoma skin lesions"
MULTIMEDIA TOOLS AND APPLICATIONS,
vol. 79,
pp. 15575
-15592
,
2020
| DOI: 10.1007/s11042-019-7717-y
Journal
![]() |
4 |
Canalini, Laura; Pollastri, Federico; Bolelli, Federico; Cancilla, Michele; Allegretti, Stefano; Grana, Costantino
"Skin Lesion Segmentation Ensemble with Diverse Training Strategies"
Computer Analysis of Images and Patterns,
vol. 11678,
Salerno, Italy,
pp. 89
-101
,
Sep 3-5,
2019
| DOI: 10.1007/978-3-030-29888-3_8
Conference
![]() |
5 |
Pollastri, Federico; Bolelli, Federico; Paredes, Roberto; Grana, Costantino
"Improving Skin Lesion Segmentation with Generative Adversarial Networks"
2018 IEEE 31st International Symposium on Computer-Based Medical Systems (CBMS),
vol. 2018-,
Karlstad, Sweden,
pp. 442
-443
,
Jun 18-21,
2018
| DOI: 10.1109/CBMS.2018.00086
Conference
![]() |