
Research on Embodied AI
Self-Supervised Navigation and Recounting
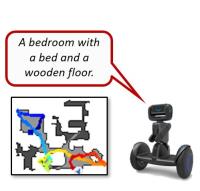
Advances in the field of embodied AI aim to foster the next generation of autonomous and intelligent robots. At the same time, tasks at the intersection of computer vision and natural language processing are of particular interest for the community, with image captioning being one of the most active areas. By describing the content of an image or a video, captioning models can bridge the gap between the black-box architecture and the user. In this project, we propose a new task at the intersection of embodied AI, computer vision, and natural language processing, and aim to create a robot that can navigate through a new environment and describe what it sees. We call this new task Explore and Explain since it tackles the problem of joint exploration and captioning. In this schema, the agent needs to perceive the environment around itself, navigate it driven by an exploratory goal, and describe salient objects and scenes in natural language. Beyond navigating the environment and translating visual cues in natural language, the agent also needs to identify appropriate moments to perform the explanation step.
Embodied Agents for Differences Discovery in Dynamic Environments
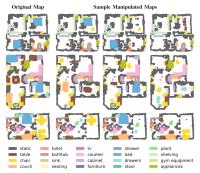
Imagine you have just bought a personal robot, and you ask it to bring you a cup of tea. It will start roaming around the house while looking for the cup. It probably will not come back until some minutes, as it is new to the environment. After the robot knows your house, instead, you expect it to perform navigation tasks much faster, exploiting its previous knowledge of the environment while adapting to possible changes of objects, people, and furniture positioning. As agents are likely to stay in the same place for long periods, such information may be outdated and inconsistent with the actual layout of the environment. Therefore, the agent also needs to discover those differences during navigation.
Acquisition of 3D Environments for Robotic Navigation
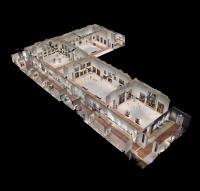
Matterport technology allows to create a “digital twin” of a physical indoor space. Thanks to the Matterport Pro2 camera, it is easy to acquire 3D information from an environment and to create a virtual space in which to train embodied agents. Thanks to this, the recently proposed Matterport3D dataset of spaces has attracted a lot of interest form the research community. Our aim is to acquire new environments, and we are particularly interested in places related with Italian Cultural Heritage. In this project, we present the 3D reconstruction of the Galleria Estense of Modena.
Embodied Vision-and-Language Navigation
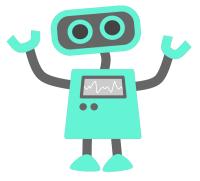
Effective instruction-following and contextual decision-making can open the door to a new world for researchers in embodied AI. Deep neural networks have the potential to build complex reasoning rules that enable the creation of intelligent agents, and research on this subject could also help to empower the next generation of collaborative robots. In this scenario, Vision-and-Language Navigation (VLN) plays a significant part in current research. This task requires to follow natural language instructions through unknown environments, discovering the correspondences between lingual and visual perception step by step. Additionally, the agent needs to progressively adjust navigation in light of the history of past actions and explored areas. Even a small error while planning the next move can lead to failure because perception and actions are unavoidably entangled; indeed, "we must perceive in order to move, but we must also move in order to perceive". For this reason, the agent can succeed in this task only by efficiently combining the three modalities - language, vision, and actions.