
Research on Multimedia and Big Visual Data
Video Captioning with Naming
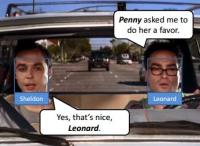
Current approaches for movie description lack the ability to name characters with their proper names, and can only indicate people with a generic "someone" tag. We developed two contributions towards the development of video description architectures with naming capabilities: firstly, we collected and released an extension of the popular Montreal Video Annotation Dataset in which the visual appearance of each character is linked both through time and to textual mentions in captions. We annotated, in a semi-automatic manner, a total of 63k face tracks and 34k textual mentions on 92 movies. Moreover, to underline and quantify the challenges of the task of generating captions with names, we presented different multi-modal approaches to solve the problem on already generated captions.
Video matching and retrieval
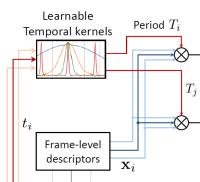
We tackle the task of retrieving and aligning similar video instances. This problem arises in different applications such as copy detection, particular event detection, video editing and re-purposing. In the literature, one can distinguish the methods offering temporal alignment and those discarding the time information, typically through temporal pooling operations. In our work, we consider the temporal matching kernel (TMK). This representation consists of complementary periodic encodings of a sequence of frames into a fixed-sized representation. It provides both an accurate matching and alignment hypothesis, and outperforms existing approaches in terms of alignement accuracy.
Scene detection in Broadcast Videos
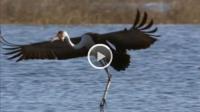
Nowadays there is a strong interest in the re-use of video content coming from major broadcasting networks, which have been producing high quality edited videos for popular science purposes, such as documentaries and similar programs. Unfortunately, re-using videos in ones own presentations or video aided lectures is not an easy task, and requires video editing skills and tools, on top of the difficulty of finding the parts of videos which effectively contain the specific content the instructor is interested in. Story detection has been recognized as a tool which effectively may help in this situation, going beyond frames and even beyond simple editing units, such as shots. The task is to identify coherent sequences in videos, without any help from the editor or publisher. Our final goal is an improved access to broadcast video footage and a possible re-use of the huge available video content with the direct management of user-selected video-clips.
Deep Learning in videos

Despite the achievements of Deep Learning on images, text, and audio data, it is still not clear how these techniques can be successfully applied to video-related tasks, like video summarization, event detection and video concept detection. In this setting, the main goal of our research is to develop and test new Deep Learning algorithms for Temporal Video Segmentation and Concept Detection in videos.
Temporal video segmentation is a well-established problem in video analysis, and aims at organizing a video into groups of frame sequences according to some coherency criterion. In case of edited video, frames are grouped into shots (sequences of frames taken by the same camera) and shots can in turn be grouped into semantically coherent segments, which are also called stories, since they often are story telling. Since the resulting segments can be automatically tagged and annotated, this decomposition allows a fine-grained search and re-use of existing video archives, and is therefore of great interest both from the scientific point of view and from the industrial applications point of view.
Video Captioning
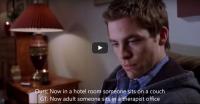
Automatically describing a video in natural language is an important challenge for computer vision and machine learning. This task, called video captioning, is a crucial achievement towards machine intelligence and also the support of a number of potential applications. Indeed, bringing together vision and language, video captioning can be leveraged for video retrieval, to enhance content search on video sharing and streaming platforms, as well as to generate automatic subtitles and to help visually impaired people to get an insight of the content of a video
Class Specific Segmentation
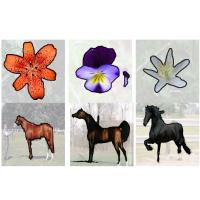
In this work we address the task of learning how to segment a particular class of objects, by means of a training set of images and their segmentations. In particular we propose a method to overcome the extremely high training time of a previously proposed solution to this problem, Kernelized Structural Support Vector Machines.We employ a one-class SVM working with joint kernels to robustly learn significant support vectors (representative image-mask pairs) and accordingly weight them to build a suitable energy function for the graph cut framework. We report results obtained on two public datasets and a comparison of training times on different training set sizes.
Garment Selection and Color Classification
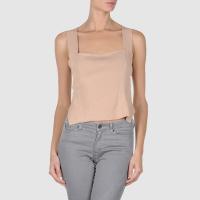
Internet shopping has grown incredibly in the last years, and fashion created an interesting application field for image understanding and retrieval, since hundreds of thousands images of clothes constitute a challenging dataset to be used for automatic or semi-automatic segmentation strategies, color analysis, texture analysis, similarity retrieval, automatic piece of clothing classification and so on. We addressed the problem of automatic segmentation, color retrieval and classification of fashion garments.
Egocentric Video Summarization
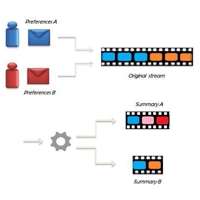
Typical egocentric video summarization approaches have dealt with motion analysis and social interaction without considering that different final users might be interested in preserving only scenes from the original video according to their specific preferences. In this paper we have proposed a new method for personalized video summarization of cultural experiences with the goal of extracting from the streams only the scenes corresponding to a user's specific topics request, chosen among high visual quality shots, identified as the ones in which it's possible to deduce camera's wearer attention behaviour pattern.
Face Recognition in News Streams
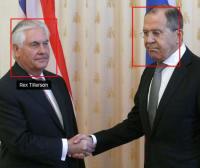
The goal of the project is to identify the foreign ministers of the G7/G20 group into the images of the daily news. The problem has been divided in two parts: on the one hand the daily main news and the relative images retrieval, on the other hand the face detection and the face recognition.