
Research on Videosurveillance and HBU
Human-Robot Interaction
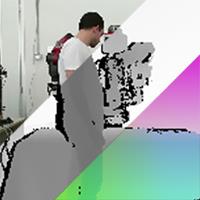
Collaborative robots, or cobots, have entered the automation market for several years now, achieving a rather rapid and wide diffusion. The Human-Robot Interaction (HRI) and Human-Robot Collaboration (HRC) paradigms still have unexplored potential and challenges not yet fully investigated and solved.
Among others, the knowledge of the instantaneous pose of robots and humans in a shared workspace is a key element to set up an effective and fruitful collaboration. The pose estimation problem opens up to new applications, ranging from solutions for the safety of the interaction to behavior analysis.
Despite robots usually provide their encoder status through dedicated communication channels, enabling the use of forward kinematics, an external method is desirable in certain cases where the robot controller is not accessible. In particular, aiming to have an end-to-end system which predicts the pose of both robots and humans, a method based on an external camera view of a collaborative scene is more suitable.
3D Human Pose Estimation from Depth Maps
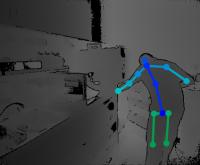
Human Pose Estimation is a fundamental task for many applications in the Computer Vision community. Although it is a widely developed area in the 2D domain, i.e. color images, few literature works aim to produce precise skeletons using different types of data. Our research acitivity is based on the use of depth image, i.e. depth-maps, to exploit the depth values, i.e. the distance from the camera, to obtain accurate 3D skeletons in the camera coordinate system.
Soccer Event Spotting
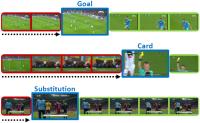
The recently proposed action spotting task consists in finding the exact timestamp in which an event occurs. This task fits particularly well for soccer videos, where events correspond to salient actions strictly defined by soccer rules (a goal occurs when the ball crosses the goal line). We devise a lightweight and modular network for action spotting, which can simultaneously predict the event label and its temporal offset using the same underlying features. We enrich our model with two training strategies: the first one for data balancing and uniform sampling, the second for masking ambiguous frames and keeping the most discriminative visual cues.
GAN4Surveillance: Generative Adversarial Networks for Attribute Classification
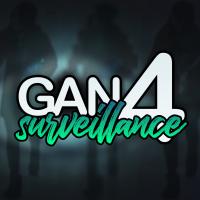
Security is of fundamental importance in a world where terrorist attacks are steadily increasing. Governments and agencies face these realities every day, but not always the means at their disposal are sufficient to effectively prevent those attacks. The security area uses many science and engineering fields, and many are the areas of study available. This research activity is focused on the problem of attribute classification (such as age, sex, etc.) and items (backpacks, bags, etc.) of people through security cameras. Computer Vision based Deep Learning techniques and generative models are exploited to address this problem in an automatic fashion. We explore the generalization capability of adversarial networks to enhance people image resolution and to hallucinate occluded body parts.
Learning to Detect and Track Visible and Occluded Body Joints in a Virtual World
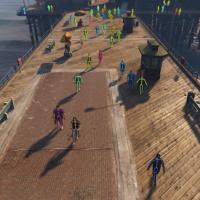
Multi-People Tracking in an open-world setting requires a special effort in precise detection. Moreover, temporal continuity in the detection phase gains more importance when scene cluttering introduces the challenging problems of occluded targets. For the purpose, we propose a deep network architecture that jointly extracts people body parts and associates them across short temporal spans.
Our model explicitly deals with occluded body parts, by hallucinating plausible solutions of not visible joints. We propose a new end-to-end architecture composed by four branches (visible heatmaps, occluded heatmaps, part affinity fields and temporal affinity fields) fed by a time linker feature extractor. To overcome the lack of surveillance data with tracking, body part and occlusion annotations we created the vastest Computer Graphics dataset for people tracking in urban scenarios by exploiting a photorealistic videogame. It is up to now the vastest dataset (about 500.000 frames, more than 10 million body poses) of human body parts for people tracking in urban scenarios.
Our architecture trained on virtual data exhibits good generalization capabilities also on public real tracking benchmarks, when image resolution and sharpness are high enough, producing reliable tracklets useful for further batch data association or re-id modules.
Duke Imagelab Multi-Target, Multi-Camera Tracking Project
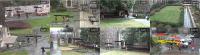
DukeMTMC aims to accelerate advances in multi-target multi-camera tracking. It provides a tracking system that works within and across cameras, a new large scale HD video data set recorded by 8 synchronized cameras with more than 7,000 single camera trajectories and over 2,000 unique identities, and a new performance evaluation method that measures how often a system is correct about who is where.
Spotting Prejudice
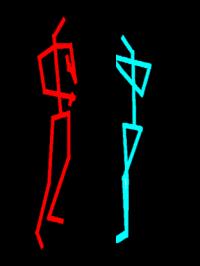
Despite prejudice cannot be directly observed, nonverbal behaviours provide profound hints on people inclinations. In this study, we use recent sensing technologies and machine
learning techniques to automatically infer the results of psychological questionnaires frequently used to assess implicit prejudice. In particular, we recorded 32 students discussing
with both white and black collaborators. Then, we identified a set of features allowing automatic extraction and measured their degree of correlation with psychological scores. Results
confirmed that automated analysis of nonverbal behaviour is actually possible thus paving the way for innovative clinical
tools and eventually more secure societies.
Action and Gesture Recognition for Human Computer Interaction

This research activity handles the problems of people action and gesture recognition. In particular, we are developing a complete framework for Human Computer Interaction (HCI), where custom gestures can be adopted by each user. Continuous gesture recognition, one-shot learning, transfer learning algorithms are taken into account.
HCI is the discipline that studies models and techniques for the interaction between people and computers. Its historical evolution starts in the ‘70s when Command Line Interfaces (CLI) were created. Although these devices are quick, they are difficult to use, because of their mnemonic component, i.e. users have to remember the right and precise command to interact with the computer. In the ‘80s Graphical User Interfaces (GUI) were developed: they are more user friendly than CLI and introduce new devices (like mouse) and metaphors (like window, drag-and-drop, and desktop). Natural User Interfaces (NUI) have been conceived in the ‘90s; they are intuitive and invisible because users do not need any material device to interact with the computer, they just perform natural actions with their body, their natural and innate language.
For these reasons, NUIs require systems that are able to automatically detect and recognize actions and gestures in a video stream. They have recently got prestige thanks to new low cost technologies that allow easily detecting and precisely tracking human body joints in a 3D space.
Group Detection and Crowd Analysis
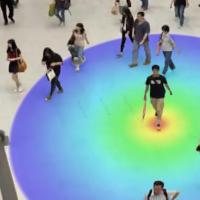
Behavior analysis will play a central role in future video surveillance systems as research on this topic has been revealing promising in helping to discover public safety risks or predict crimes. Nevertheless, trying to understand complex interactions in the scene just by looking at each individual separately is unrealistic, due to the inherent social nature of human behavior. This is because those interactions do not occur at an individual level nor at a crowd level, but they typically involve small subsets of people, namely groups. We thus believe future challenges will reside in enhancing action analysis by considering social interactions among small gathering of people sharing a common goal, to this end group detection becomes a mandatory step for modern crowd surveillance systems.
Multiple People Tracking
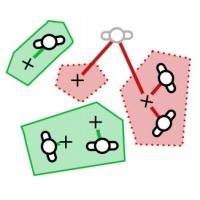
Multiple Target Tracking is an important task within the field of computer vision. The proliferation of high-powered computers, the availability of high quality and inexpensive video cameras, and the increasing need for automated video analysis has generated a great deal of interest in tracking algorithms. The problem is often addressed in a paradigm named tracking-by-detection, where detections are given ahead of time and tracking purpose is to merge this detections into separate identities. The real challenge in Multi Target Tracking is how to deal with noisy detections (miss and false detections) and with long occlusions. In this work we leveraged on cognitive psychology studies to develop a human-inspired model.
People Re-identification
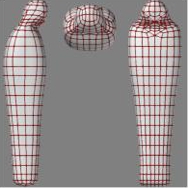
People re-identification aims at finding multiple instance of the same person on images or videos based on appearance features. Imagelab attempted to solve the re-identification problem by means of 3D body models, that provide a spatial support for the appearance features.
ViSOR - Video Surveillance Online Repository
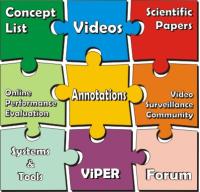
ViSOR contains a large set of multimedia data and the corresponding annotations. The repository has been conceived as a support tool for different research projects.
Together with the videos, ViSOR contains metadata annotation, both manually annotated ground-truth data and automatically obtained outputs of a particular system. In such a manner, the users of the repository are able to perform validation tasks of their own algorithms as well as comparative activities. ViSOR also contains two datasets for people Reidentification: 3DPES and SARC3D.
People trajectory analysis and anomaly detection
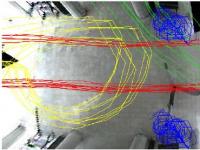
People trajectory analysis is a recurrent task in many pattern recognition applications, such as surveillance, behavior analysis, video annotation, and many others. We develop a new framework for analyzing trajectory shape, invariant to spatial shifts of the people motion in the scene.
People Tracking From Multiple Cameras

Outdoor surveillance is one of the most attractive application of video processing and analysis. Robust algorithms must be defined and tuned to cope with the non-idealities of outdoor scenes. For instance, in a public park, an automatic video surveillance system must discriminate between shadows, reflections, waving trees, people standing still or moving, and other objects. Visual knowledge coming from multiple cameras can disambiguate cluttered and occluded targets by providing a continuous consistent labeling of tracked objects among the different views.
Video Action Detection
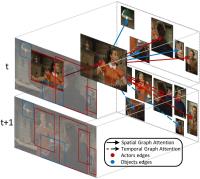
Video action detection requires an algorithm to detect and classify human actions in a video clip. Tackling the problem requires to address challenges that lie at the intersection between low-level and high-level video understanding. Firstly, fine-grained and discriminative spatio-temporal features are needed to represent video chunks in a compact and manageable form.
On the other hand, detecting and understanding human actions is not just a matter of extracting middle-level features, and demands for more high-level reasoning. We devise a high-level module for video action detection which considers interactions between different people in the scene and interactions between actors and objects. Further, we also take into account long-range temporal dependencies by connecting consecutive clips during learning and inference.
Trajectory Prediction
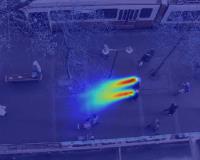
Anticipating human motion in crowded scenarios is essential for developing intelligent transportation systems, social-aware robots, and advanced video-surveillance applications. An important aspect of such a task is represented by the inherently multi-modal nature of human paths which makes socially-acceptable multiple futures when human interactions are involved.
INSECTT Anomaly detection SETA Dataset
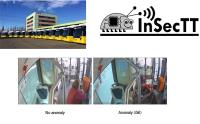
Long, untrimmed real-world surveillance videos with 11 realistic anomalies recorded with a series of CCTV cameras placed inside SETA buses.
Dataset Hightlights:
- 58 different bus rides from 2020.
- up to 5 cameras with multiple angles
- 31 hours of video
- Divided in 182 sequence
The dateaset is up to date the largest dataset in the world about public transports anomalies in a single setting(Scenario). It uses non-crowdsourced data. Data are acquired on field in the operative scenario.