
Facebook AI Research Partnership
“Structured Deep Networks for video understanding”
Imagelab is devoted to define new deep learning architectures for tackling two main problems in computer vision:
-
Video annotation and semantic concept extraction on edited broadcasting videos (in cooperation with RAI)
-
Temporal saliency in videos for context and actions understanding in surveillance, video broadcasting and automotive

To this end, we are studying and testing innovative deep learning architectures by means of structured predictors and focusing on temporal data. At a glance, our research lines can be summarized as following:
Video annotation and semantic concept extraction
One of the main goal of our research is to develop and test new Deep Learning algorithms for Temporal Video Segmentation and Concept Detection in videos. We developed a new approach to Temporal Video Segmentation which was able to beat all existing approaches on benchmark datasets, and which relies on a multimodal Deep Neural Network that combines static appearance, features from the video transcript, and concept detection in a metric learning fashion. The core of the algorithm is a concept detection strategy which can analyse the video transcript and build classifiers for relevant concepts “on the fly”, exploiting the Imagenet database. Our research in the next months will include a complete refinement of this approach, as well as the development of new algorithms for concept detection and one shot learning in videos. We also plan to perform theoretical studies on Convolutional Neural Networks and Recurrent Neural Networks for structured prediction. Training and fine-tuning of these architectures will be performed on large-scale video and image datasets (like YFCC-100M, SPORTS-1M, ILSVRC-2012), thus requiring adequate computational capacities.
Temporal saliency in videos for context and actions understanding
One line of research consists in transferring the current results on pixel level segmentation to salient objects. This is indeed a well defined task for images but still understudied in videos. Saliency is undoubtedly influenced by both the observer task and temporal coherence. The underlying idea of our research is to exploit 3D CNN to account for temporal features and, at the same time, to add a structured predictor CRF to the Deep NN in order to account for objectness and semantics. We aim at testing on recent dataset for both autonomous driving application, by this end we recently release a driver fixations dataset, and on recent video activity datasets like the “Action in the eye” dataset. In the current research panorama we believe this is an interesting line of research with multiple applications ranging from comprehending the mechanism behind attention and actions to providing summaries of videos by means of interesting objects alone and their activities.
A relevant scientific impact is expected, both in international computer vision and multimedia conferences and in top class journals, like IEEE Transactions on PAMI, IJCV, Pattern Recongition, CVPR, ICCV, ACM Multimedia, NIPS, ICLR.
Contacts and required details
-
Primary liaison for the institution: Prof. Rita Cucchiara, Director of Imagelab and Softech-ICT
-
Primary IT contact: Prof. Costantino Grana, Imagelab, Softech-ICT.
-
Other IT contacts: Dr. Simone Calderara (Assistant Professor), Ing. Lorenzo Baraldi, Ing. Stefano Alletto, Ing. Francesco Solera (PhD Students).
- Number of researchers conducting AI or machine learning research: Softech-ICT is an interdepartmental research centre, composed by more than 50 researchers. About 40% of them are working in machine learning and AI applied to computer vision, pattern recognition, big data and complex systems. Among them, the ImageLab research group (www.imagelab.unimore.it) is composed by five staff people and about 12 researchers (8 PhD students and post docs) working on deep learning and machine learning for computer vision and video analysis.
Publications
1 |
Baraldi, Lorenzo; Douze, Matthijs; Cucchiara, Rita; Jégou, Hervé
"LAMV: Learning to align and match videos with kernelized temporal layers"
2018 IEEE/CVF Conference on Computer Vision and Pattern Recognition,
Salt Lake City, UT, USA, USA,
pp. 7804
-7813
,
June 18-22,
2018
| DOI: 10.1109/CVPR.2018.00814
Conference
![]() |
2 |
Baraldi, Lorenzo; Grana, Costantino; Cucchiara, Rita
"Recognizing and Presenting the Storytelling Video Structure with Deep Multimodal Networks"
IEEE TRANSACTIONS ON MULTIMEDIA,
vol. 19,
pp. 955
-968
,
2017
| DOI: 10.1109/TMM.2016.2644872
Journal
![]() |
3 |
Baraldi, Lorenzo; Grana, Costantino; Cucchiara, Rita
"Hierarchical Boundary-Aware Neural Encoder for Video Captioning"
Computer Vision and Pattern Recognition (CVPR), 2017 IEEE Conference on,
vol. 2017-,
Honolulu, Hawaii,
pp. 3185
-3194
,
July, 22-25,
2017
| DOI: 10.1109/CVPR.2017.339
Conference
![]() |
Project Info
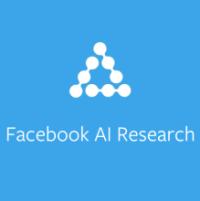