
Learning to Detect and Track Visible and Occluded Body Joints in a Virtual World
Multi-People Tracking in an open-world setting requires a special effort in precise detection. Moreover, temporal continuity in the detection phase gains more importance when scene cluttering introduces the challenging problems of occluded targets. For the purpose, we propose a deep network architecture that jointly extracts people body parts and associates them across short temporal spans.
Our model explicitly deals with occluded body parts, by hallucinating plausible solutions of not visible joints. We propose a new end-to-end architecture composed by four branches (visible heatmaps, occluded heatmaps, part affinity fields and temporal affinity fields) fed by a time linker feature extractor. To overcome the lack of surveillance data with tracking, body part and occlusion annotations we created the vastest Computer Graphics dataset for people tracking in urban scenarios by exploiting a photorealistic videogame. It is up to now the vastest dataset (about 500.000 frames, more than 10 million body poses) of human body parts for people tracking in urban scenarios.
Our architecture trained on virtual data exhibits good generalization capabilities also on public real tracking benchmarks, when image resolution and sharpness are high enough, producing reliable tracklets useful for further batch data association or re-id modules.
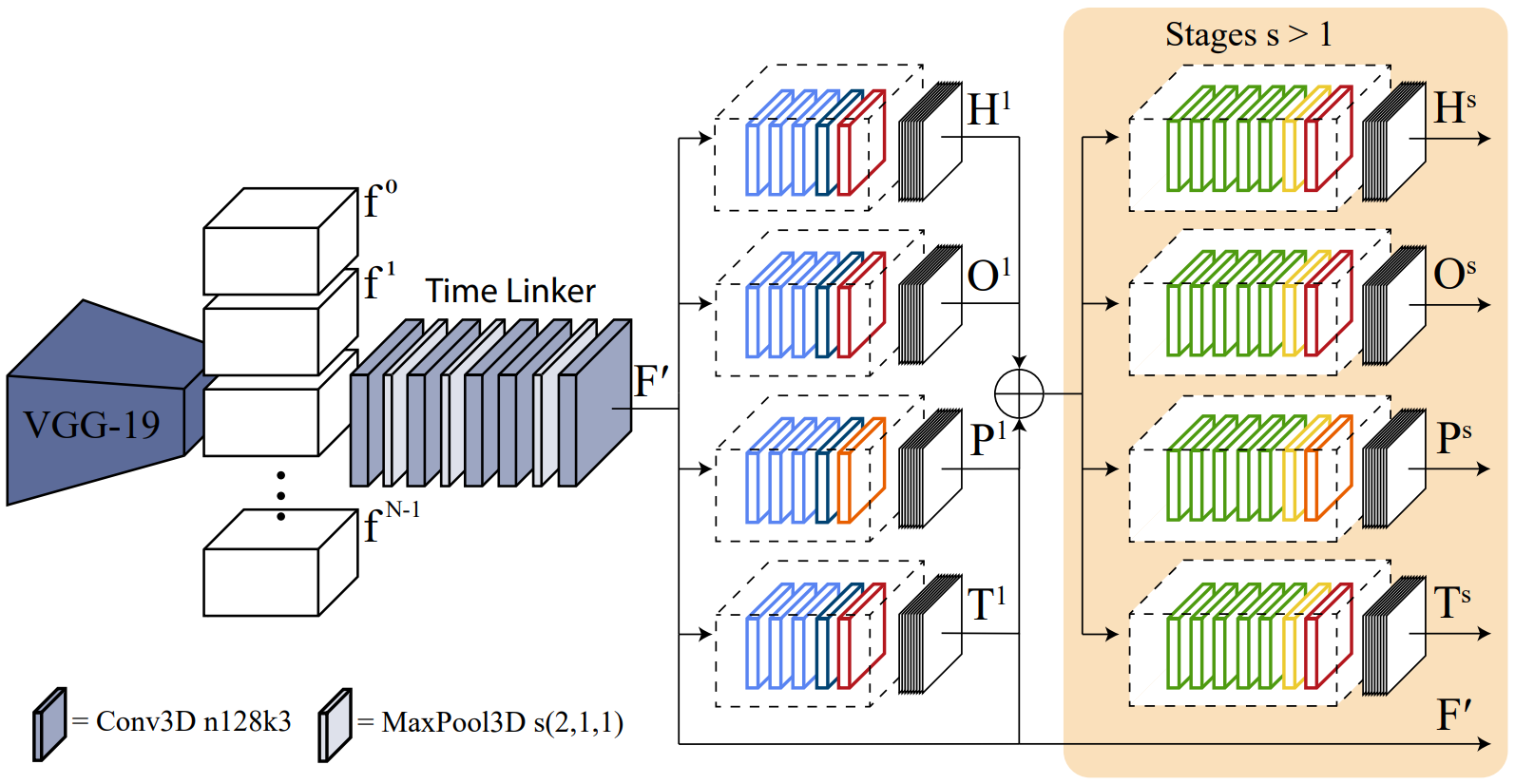
Multi-People Tracking (MPT) is one of the most established fields in computer vision. It has been recently fostered by the availability of comprehensive public benchmarks and data. Often, MPT approaches have been casted in the tracking by detection paradigm where a pedestrian detector extracts candidate objects and a further association mechanism arranges them in a temporally consistent trajectory. Nevertheless, in the last years several researchers raised the question on whether these two phases would be disentangled or considered two sides of the same problem.
The strong influence between detection accuracy and tracking performance suggests considering detection and tracking as two parts of a unique problem that should be addressed end-to-end at least for short-term setups. In this work, we advocate for an integrated approach between detection and short-term tracking that can serve as a proxy for more complex association method either belonging to the tracking or re-id family of techniques.
To this aim, we propose:
- an end-to-end deep network, called THOPA-net (Temporal Heatmaps and Occlusions based body Part Association) that jointly locates people body parts and associates them across short temporal spans. This is achievable with modern deep learning architectures that exhibit terrific performance in body part location but, mostly, neglect the temporal contribution. For the purpose, we propose a bottom-up human pose estimation network with a temporal coherency module that jointly enhances the detection accuracy and allows for short-term tracking;
- an explicit method for dealing with occluded body parts that exploits the capability of deep networks of hallucinating feasible solutions;
- a massive computer graphics dataset, namely JTA (Joints Tracking Annotated dataset), that simulates realistic people tracking scenarios in a virtual world, in accordance with recent literature that testifies the advantage of disposing of virtual world proxy for several deep learning problems. Our dataset is the first of its kind for people surveillance in urban scenarios and comes with a rich automatic annotation on people body part locations and their per-frame tracking. The dataset is composed by about 500K frames and 128 different scenarios from both fixed and moving cameras and it covers the most frequent challenges of MPT in urban areas: almost 20K identities, crowded scenes with up to 60 people, 10 millions of body poses.
Results are very encouraging in their precision also in crowded scenes. Our experiments tell us that the problem is less dependent on the details or the realism of the shape than one could imagine; instead, it is more affected by the image quality and resolution that are extremely high in Computer Graphics (CG) generated datasets. Nevertheless, experiments on real MPT dataset demonstrate that with a minimal amount of fine-tuning the model can transfer positively towards real scenarios.
Publications
1 |
Fabbri, Matteo; Lanzi, Fabio; Calderara, Simone; Palazzi, Andrea; Vezzani, Roberto; Cucchiara, Rita
"Learning to Detect and Track Visible and Occluded Body Joints in a Virtual World"
Proceedings of the 15th European Conference on Computer Vision (ECCV) 2018,
vol. 11208,
Munich (Germany),
pp. 450
-466
,
September, 8-14 2018,
2018
| DOI: 10.1007/978-3-030-01225-0_27
Conference
![]() |