
Visual-Semantic Domain Adaptation in Digital Humanities
While several approaches to bring vision and language together are emerging, none of them has yet addressed the digital humanities domain, which, nevertheless, is a rich source of visual and textual data. To foster research in this direction, we investigate the learning of visual-semantic embeddings for historical document illustrations and data from the digital humanities, devising both supervised and semi-supervised approaches.
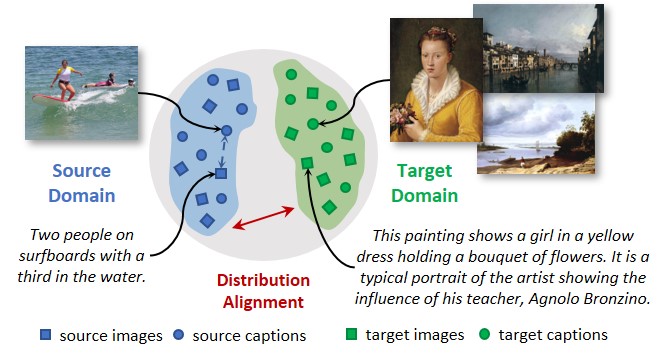
Explaining Digital Humanities by Aligning Images and Textual Descriptions
Replicating the human ability to connect Vision and Language has recently been gaining a lot of attention in the Computer Vision and the Natural Language Processing communities. This research effort has resulted in algorithms that can retrieve images from textual descriptions and vice versa, when realistic images and sentences with simple semantics are employed and when paired training data is provided. In this paper, we go beyond these limitations and tackle the design of visual-semantic algorithms in the domain of the Digital Humanities. This setting not only advertises more complex visual and semantic structures but also features a significant lack of training data which makes the use of fully-supervised approaches infeasible. With this aim, we propose a joint visual-semantic embedding that can automatically align illustrations and textual elements without paired supervision. This is achieved by transferring the knowledge learned on ordinary visual-semantic datasets to the artistic domain. Experiments, performed on two datasets specifically designed for this domain, validate the proposed strategies and quantify the domain shift between natural images and artworks.
Paper
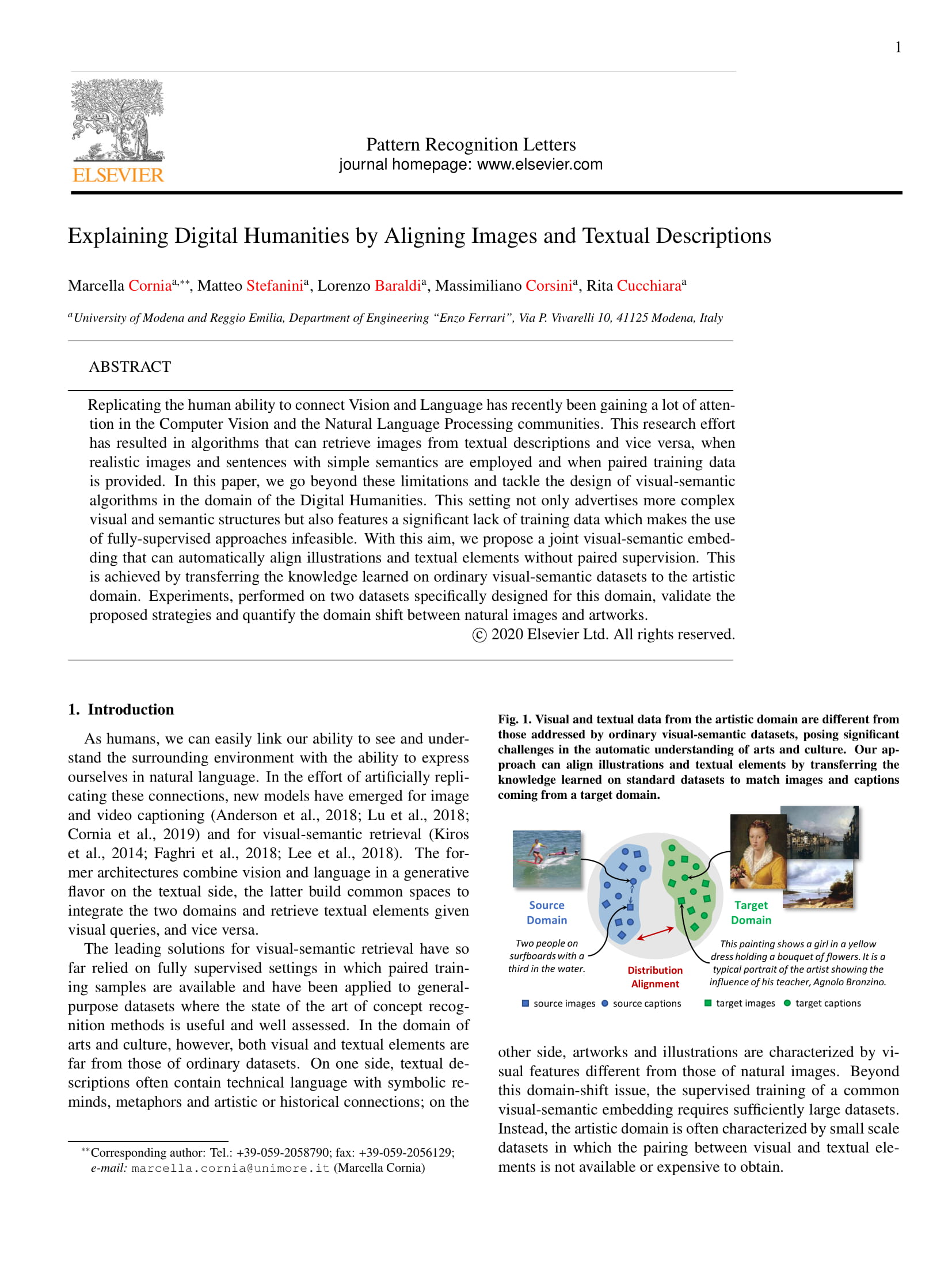
Explaining Digital Humanities by Aligning Images and Textual Descriptions
M.Cornia, M.Stefanini, L. Baraldi, M.Corsini, R.Cucchiara
Pattern Recognition Letters 2020
Artpedia: A New Visual-Semantic Dataset with Visual and Contextual Sentences
As vision and language techniques are widely applied to realistic images, there is a growing interest in designing visual-semantic models suitable for more complex and challenging scenarios. In this paper, we address the problem of cross-modal retrieval of images and sentences coming from the artistic domain. To this aim, we collect and manually annotate the \textit{Artpedia} dataset that contains paintings and textual sentences describing both the visual content of the paintings and other contextual information. Thus, the problem is not only to match images and sentences, but also to identify which sentences actually describe the visual content of a given image. To this end, we devise a visual-semantic model that jointly addresses these two challenges by exploiting the latent alignment between visual and textual chunks. Experimental evaluations, obtained by comparing our model to different baselines, demonstrate the effectiveness of our solution and highlight the challenges of the proposed dataset.
Paper
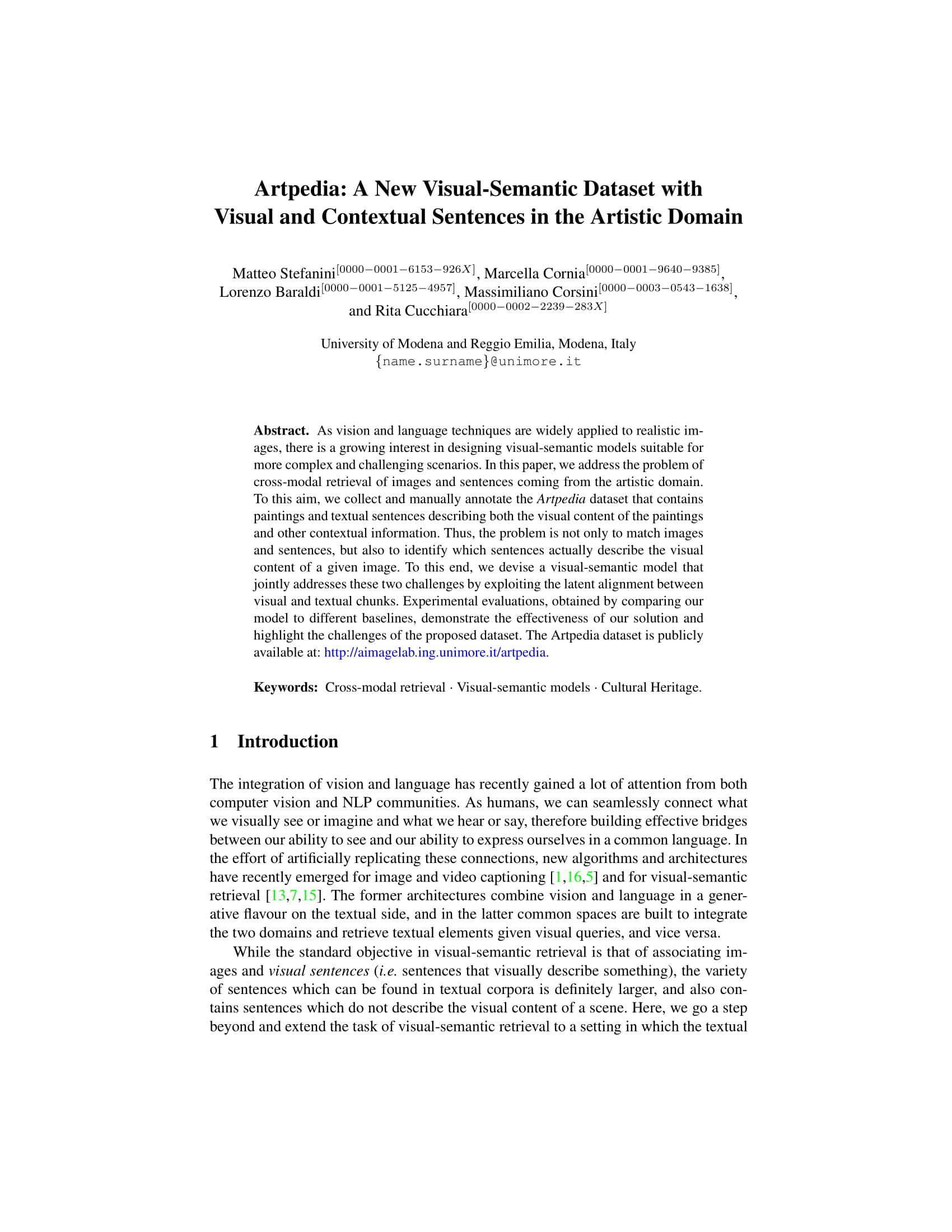
Artpedia: A New Visual-Semantic Dataset with Visual and Contextual Sentences
M.Stefanini, M.Cornia, L. Baraldi, M.Corsini, R.Cucchiara
ICIAP 2019
Aligning Text and Document Illustrations: towards Visually Explainable Digital Humanities
While several approaches to bring vision and language together are emerging, none of them has yet addressed the digital humanities domain, which, nevertheless, is a rich source of visual and textual data. To foster research in this direction, we investigate the learning of visual-semantic embeddings for historical document illustrations, devising both supervised and semi-supervised approaches. We exploit the joint visual-semantic embeddings to automatically align illustrations and textual elements, thus providing an automatic annotation of the visual content of a manuscript. Experiments are performed on the Borso d'Este Holy Bible, one of the most sophisticated illuminated manuscript from the Renaissance, which we manually annotate aligning every illustration with textual commentaries written by experts. Experimental results quantify the domain shift between ordinary visual-semantic datasets and the proposed one, validate the proposed strategies, and devise future works on the same line.
Paper
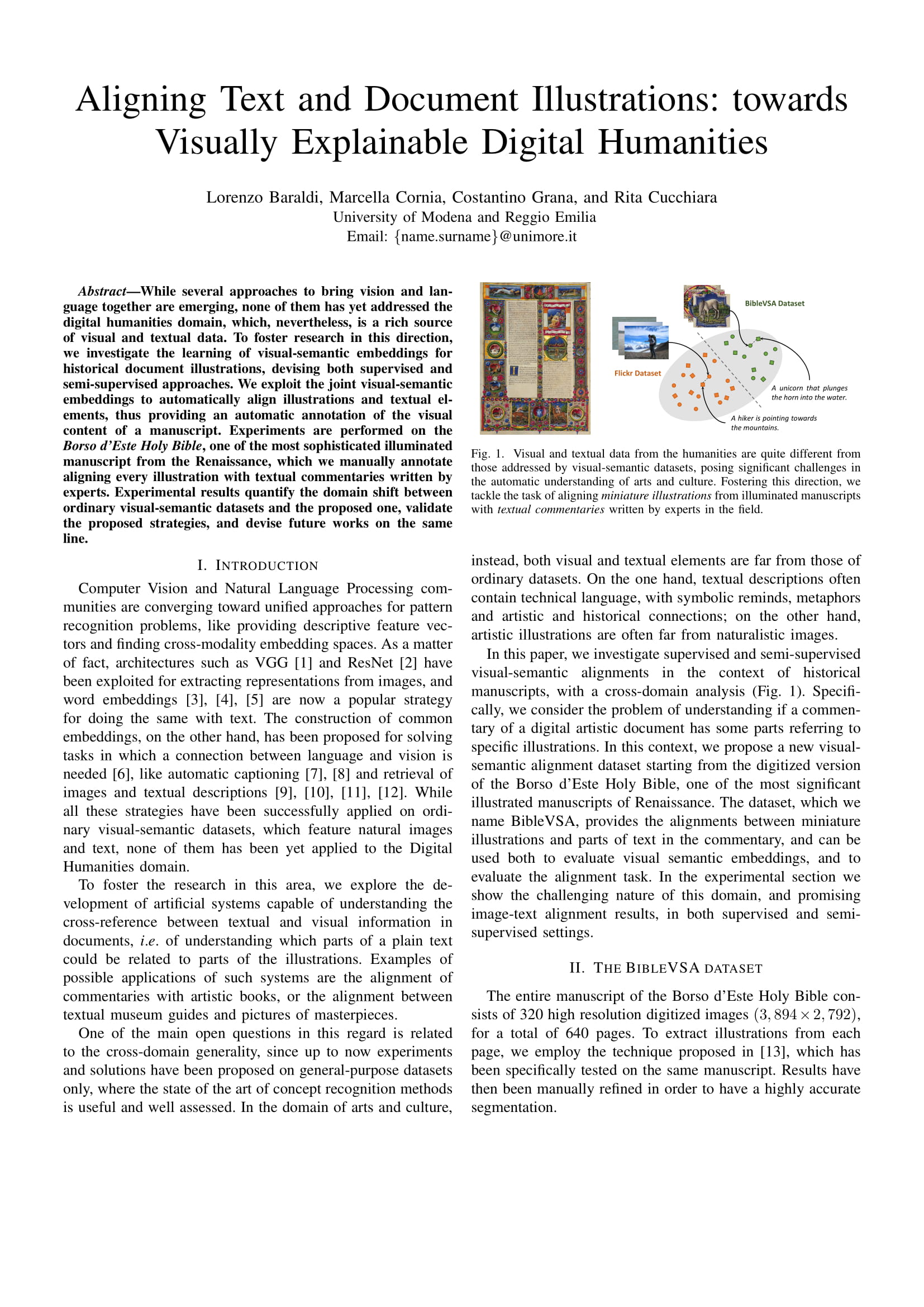
Aligning Text and Document Illustrations: towards Visually Explainable Digital Humanities
L. Baraldi, M.Cornia, C. Grana, R.Cucchiara
ICPR 2018
Publications
1 |
Cornia, Marcella; Stefanini, Matteo; Baraldi, Lorenzo; Corsini, Massimiliano; Cucchiara, Rita
"Explaining Digital Humanities by Aligning Images and Textual Descriptions"
PATTERN RECOGNITION LETTERS,
vol. 129,
pp. 166
-172
,
2020
| DOI: 10.1016/j.patrec.2019.11.018
Journal
![]() |
2 |
Stefanini, Matteo; Cornia, Marcella; Baraldi, Lorenzo; Corsini, Massimiliano; Cucchiara, Rita
"Artpedia: A New Visual-Semantic Dataset with Visual and Contextual Sentences in the Artistic Domain"
Image Analysis and Processing – ICIAP 2019,
Trento, Italy,
pp. 729
-740
,
9-13 September, 2019,
2019
| DOI: 10.1007/978-3-030-30645-8_66
Conference
![]() |
3 |
Carraggi, Angelo; Cornia, Marcella; Baraldi, Lorenzo; Cucchiara, Rita
"Visual-Semantic Alignment Across Domains Using a Semi-Supervised Approach"
Computer Vision – ECCV 2018 Workshops,
vol. 11134,
Munich, Germany,
pp. 625
-640
,
8-14 September 2018,
2019
| DOI: 10.1007/978-3-030-11024-6_47
Conference
![]() |
4 |
Baraldi, Lorenzo; Cornia, Marcella; Grana, Costantino; Cucchiara, Rita
"Aligning Text and Document Illustrations: towards Visually Explainable Digital Humanities"
Proceedings of the 24th International Conference on Pattern Recognition,
Beijing, China,
pp. 1097
-1102
,
August 20th-24th, 2018,
2018
| DOI: 10.1109/ICPR.2018.8545064
Conference
![]() |