
Embodied Vision-and-Language Navigation
Effective instruction-following and contextual decision-making can open the door to a new world for researchers in embodied AI. Deep neural networks have the potential to build complex reasoning rules that enable the creation of intelligent agents, and research on this subject could also help to empower the next generation of collaborative robots. In this scenario, Vision-and-Language Navigation (VLN) plays a significant part in current research. This task requires to follow natural language instructions through unknown environments, discovering the correspondences between lingual and visual perception step by step. Additionally, the agent needs to progressively adjust navigation in light of the history of past actions and explored areas. Even a small error while planning the next move can lead to failure because perception and actions are unavoidably entangled; indeed, "we must perceive in order to move, but we must also move in order to perceive". For this reason, the agent can succeed in this task only by efficiently combining the three modalities - language, vision, and actions.
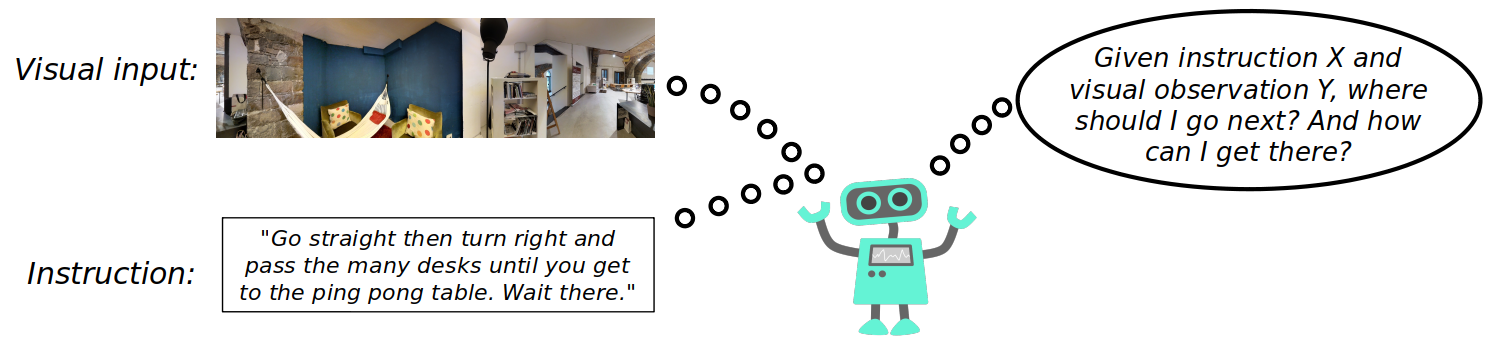
Vision-and-Language Navigation with Dynamic Convolutional Filters
In this paper, we propose to exploit dynamic convolutional filters to encode the visual information and the lingual description in an efficient way. Differently from some previous works that abstract from the agent perspective and use high-level navigation spaces, we design a policy which decodes the information provided by dynamic convolution into a series of low-level, agent friendly actions.
Results show that our model exploiting dynamic filters performs better than other architectures with traditional convolution, being the new state of the art for embodied VLN in the low-level action space. Additionally, we attempt to categorize recent work on VLN depending on their architectural choices and distinguish two main groups: we call them low-level actions and high-level actions models. To the best of our knowledge, we are the first to propose this analysis and categorization for VLN.
Paper
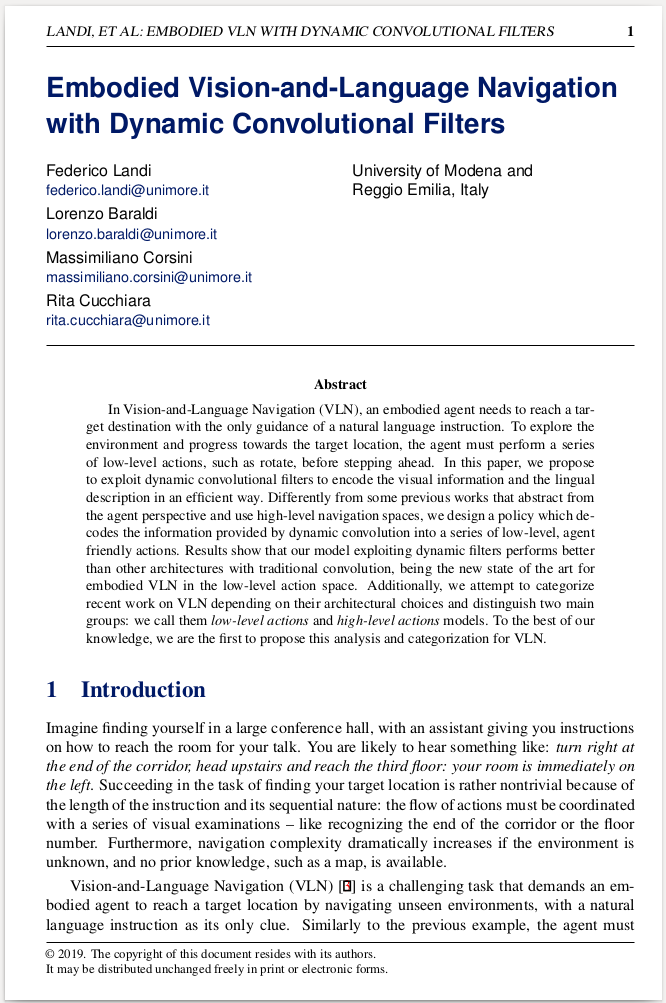
Embodied Vision-and-Language Navigation with Dynamic Convolutional Filters
F.Landi, L.Baraldi, M.Corsini, R.Cucchiara
BMVC 2019
Bibtex
If you find our work useful for your research, please cite:
@inproceedings{landi2019embodied, title={Embodied Vision-and-Language Navigation with Dynamic Convolutional Filters}, author={Landi, Federico and Baraldi, Lorenzo and Corsini, Massimiliano and Cucchiara, Rita}, booktitle={30th British Machine Vision Conference}, year={2019} }
Perceive, Transform, and Act: Multi-Modal Attention Networks for Vision-and-Language Navigation
In this paper, we strive for the creation of an agent able to tackle three key issues: multi-modality, long-term dependencies, and adaptability towards different locomotive settings. To that end, we devise "Perceive, Transform, and Act" (PTA): a fully-attentive VLN architecture that leaves the recurrent approach behind and the first Transformer-like architecture incorporating three different modalities - natural language, images, and low-level actions for the agent control. In particular, we adopt an early fusion strategy to merge lingual and visual information efficiently in our encoder. We then propose to refine the decoding phase with a late fusion extension between the agent's history of actions and the perceptual modalities. We experimentally validate our model on two datasets: PTA achieves state-of-the-art results in low-level VLN on R2R and achieves the first place in the recently proposed R4R benchmark.
Paper
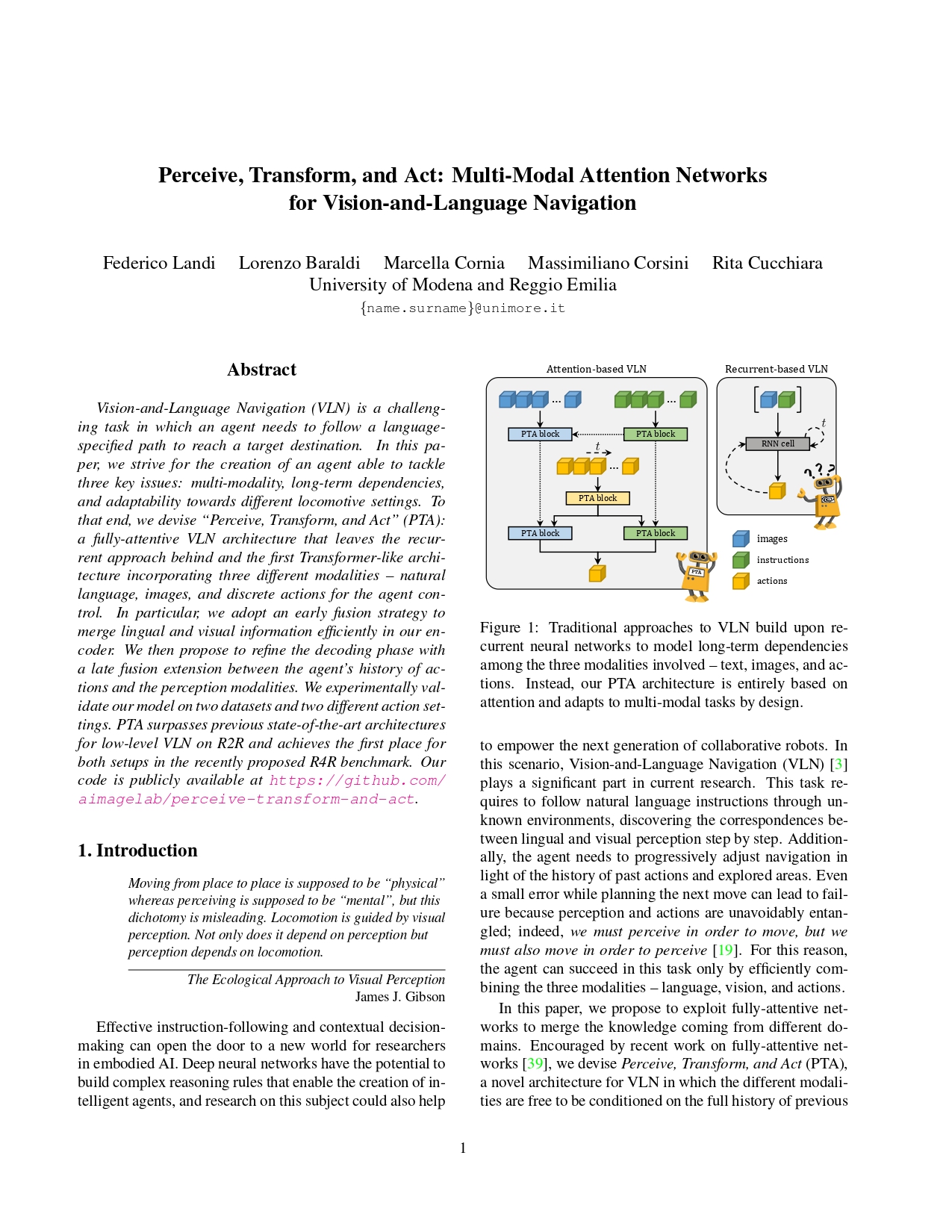
Perceive, Transform, and Act: Multi-Modal Attention Networks for Vision-and-Language Navigation
F.Landi, L.Baraldi, M.Cornia, M.Corsini, R.Cucchiara
arXiv 2019
Contacts
If you have any question, please contact the author.
Publications
1 |
Landi, Federico; Baraldi, Lorenzo; Corsini, Massimiliano; Cucchiara, Rita
"Embodied Vision-and-Language Navigation with Dynamic Convolutional Filters"
Proceedings of 30th British Machine Vision Conference,
Cardiff, UK,
pp. 1
-12
,
9th-12th September 2019,
2019
Conference
![]() |