
Decoding Gene Expression using DNA Sequence and Exploiting Post-Translational Modifications
Understanding gene expression is crucial for deciphering cellular processes and disease mechanisms. Traditional methods primarily focus on the analysis of DNA sequence data to predict gene expression levels. However, recent research has highlighted the importance of post-translational modifications (PTMs) in regulating gene expression. PTMs, such as methylation, play key roles in modulating protein activity and stability, ultimately impacting gene expression.
By incorporating PTM data, researchers can gain a deeper understanding of the regulatory mechanisms governing gene expression dynamics. Our aim is to encompass computational and experimental approaches that leverage DNA sequence data and PTM profiles to predict and interpret gene expression patterns. Decoding gene expression through the synergy of DNA sequence analysis and PTM exploitation provides a powerful framework for unraveling the complexities of gene regulation and its impact on cellular function and disease processes.
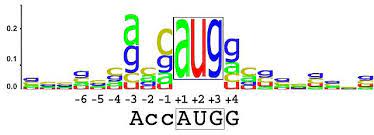