
Studying host-pathogen interaction via microscopy and Deep Learning: application to antimicrobial resistant bacteria and monoclonal antibodies discovery
The project aims to develop deep-learning techniques for analyzing biological images and videos. The study of the interaction of bacteria with the host is an integral part of vaccine design. It is usually performed using cell models and ad-hoc microscopy, often with non-scalable approaches that drastically reduce the throughput of the methodology and its application for strong antimicrobial resistance. To evaluate antibodies' effectiveness against bacterial strains, we are developing methods combining image and video analysis and machine learning/deep learning techniques. The project’s goals fulfill the national need for protocols and intelligent tools for discovering therapy resistance mechanisms and new molecules against infectious agents for developing monoclonal antibodies and vaccines.
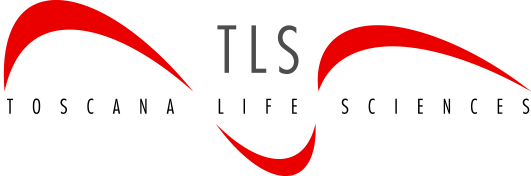