
Multi-Omic Graph Neural Networks for Cancer Prognosis and Biomarker Identification
Cancer is a multifaceted and heterogeneous disease, posing challenges in predicting its outcome due to the intricate interplay of various molecular factors. Understanding the influence of diverse biological data, including mRNA expression, DNA methylation, and copy number variations (CNVs), is crucial for enhancing prognosis and treatment strategies in cancer progression. These varied molecular data types yield valuable insights into gene regulation, genetic instability, and tumor growth, offering complementary perspectives on cancer biology.
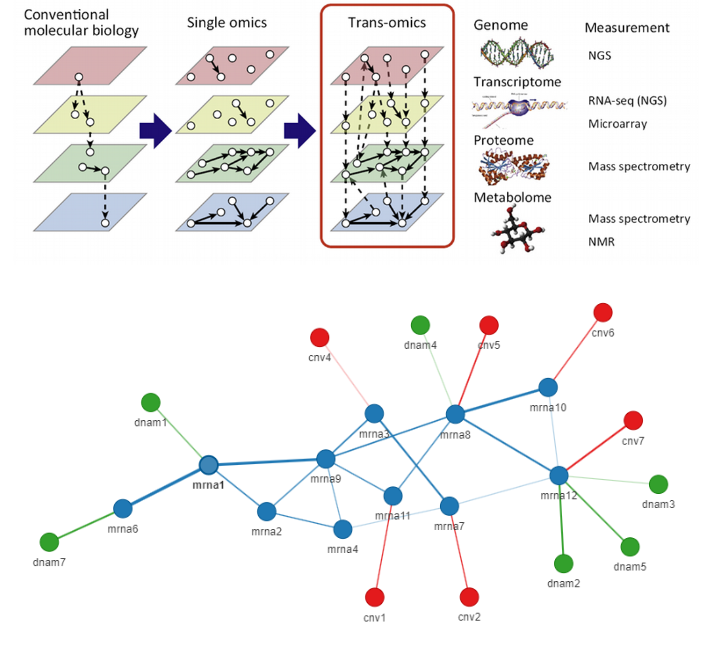
Our research is focused on the integration of multi-omic data using Graph Neural Networks (GNNs). In this approach, each patient is represented as a heterogeneous graph with multi-omic features, and the edges capture biological interactions or similarities. GNNs are adept at capturing relationships in graph-structured data, enabling the discovery of hidden patterns and potential biomarkers that impact cancer outcomes. This multi-omic graph framework has the potential to enhance the accuracy of cancer prognosis by taking into account the complex interplay between various molecular layers.
A Gentle Introduction to Deep Learning for Graphs, Bacciu et al.
The interpretability of graph structures is a crucial element of this project, allowing for the extraction of biologically meaningful insights from the model. By pinpointing crucial molecular interactions and features, the framework seeks to offer a deeper understanding of cancer mechanisms and contribute to personalized medicine.