
DriverAttention - Monitoring the car driver’s attention with multisensory systems, computer vision and machine learning
Driver inattention is one of the most important factor in highway crashes. The National Highway Traffic Safety Administration reports that about 25% of police-reported crashes involves driver inattention. Driver inattention can be classified into two main categories:distraction and fatigue.
In turn, distraction is divided in three areas: Visual Distraction (e.g. driver's gaze is not on the road), Cognitive Distraction (e.g. driver is not focused into driving activity) and Manual Distraction, that can be classified as Auditory Distraction (e.g. driver is talking at the phone) and Bio-mechanical Distraction (e.g. driver's hands are not on the steering wheel). Head pose analysis is a key aspect to investigate this kind of driver distractions and fatigue: for example, driver's head movements and cognitive distraction are correlated during driving activity. Besides, head pose and eye location information are combined to estimate the driver gaze estimation. Other literature works reveal that driver inspection patterns on the forward view are influenced by cognitive and visual distraction.
Furthermore, many car companies have implemented and installed own monitoring systems for driver posture, behavior and attention analysis, proving the increase of interest in this research field.
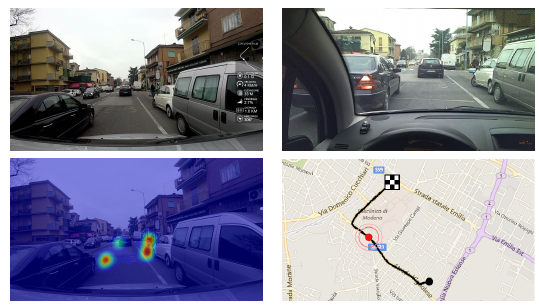
Automotive context is a challenging environment and imposes some requirements on installed systems inside a car cockpit. A fundamental aspect is the light invariance: systems have to be reliable even in presence of dramatic light changes (day, night, tunnels, bad weather conditions).
Car companies tackle this problem with the adoption of near infrared devices, such as depth cameras, that are less prone to fail in these conditions in respect to standard RGB cameras. Also the non-invasivity of the systems is a key aspect: driver's movements and gaze must be not impeded during driving activity and hardware devices have to be integrated inside the limited space of the car cockpit. Several solutions have been proposed and are based on Physiological Signals (electroencephalography, electrocardiography and electromyography) collected with sensors placed usually inside the steering wheel or the car seat, Vehicle Signals (parameters acquired from the car bus) and Physical Signals based on image acquisition and elaboration: in this paradigm vision-based approaches are probably the best solution. Besides, the presence of several occlusion and the dynamism of the driver body inside the cockpit require robust detection and classification algorithms. Finally, eal time performance are necessary to detect anomalies and immediately product warning alarms. [from IV 2017 symposium]
Publications
1 |
Venturelli, Marco; Borghi, Guido; Vezzani, Roberto; Cucchiara, Rita
"Deep Head Pose Estimation from Depth Data for In-car Automotive Applications"
Proceedings of the 2nd International Workshop on Understanding Human Activities through 3D Sensors,
vol. 10188,
Cancun (Mexico),
pp. 74
-85
,
Dec 4 , 2016,
2018
| DOI: 10.1007/978-3-319-91863-1_6
Conference
![]() |
2 |
Borghi, Guido; Venturelli, Marco; Vezzani, Roberto; Cucchiara, Rita
"POSEidon: Face-from-Depth for Driver Pose Estimation"
Proceedings of the IEEE Conference on Computer Vision and Pattern Recognition (CVPR),
vol. 2017-,
Honolulu, Hawaii,
pp. 5494
-5503
,
July, 22-25, 2017,
2017
| DOI: 10.1109/CVPR.2017.583
Conference
![]() |
3 |
Venturelli, Marco; Borghi, Guido; Vezzani, Roberto; Cucchiara, Rita
"From Depth Data to Head Pose Estimation: a Siamese approach"
Proceedings of the 12th International Joint Conference on Computer Vision, Imaging and Computer Graphics Theory and Applications (VISAPP),
vol. 5,
Porto, Portugal,
pp. 194
-201
,
27 february - 1 march, 2017,
2017
| DOI: 10.5220/0006104501940201
Conference
![]() |
4 |
Alletto, Stefano; Palazzi, Andrea; Solera, Francesco; Calderara, Simone; Cucchiara, Rita
"DR(eye)VE: a Dataset for Attention-Based Tasks with Applications to Autonomous and Assisted Driving"
IEEE Internation Conference on Computer Vision and Pattern Recognition Workshops (CVPRW),
Las Vegas,
2016,
2016
| DOI: 10.1109/CVPRW.2016.14
Conference
![]() |