
Video matching and retrieval
We tackle the task of retrieving and aligning similar video instances. This problem arises in different applications such as copy detection, particular event detection, video editing and re-purposing. In the literature, one can distinguish the methods offering temporal alignment and those discarding the time information, typically through temporal pooling operations. In our work, we consider the temporal matching kernel (TMK). This representation consists of complementary periodic encodings of a sequence of frames into a fixed-sized representation. It provides both an accurate matching and alignment hypothesis, and outperforms existing approaches in terms of alignement accuracy.
An advantage of TMK is that it disentangles the visual and temporal aspects while keeping the temporal consistency. Our proposal revists temporal match kernels in the context of a neural network. More specifically, we propose a temporal layer inspired by TMK. The design is modified and the parameters are learned with a supervision signal that takes into account both the matching quality and the precision of the alignement. This is in contrast to the original technique, where the parameters are hand-crafted by a choice of a specific kernel (Von Mises). To train our layer, we adopt a temporal proposal strategy providing both positive and negative examples. The learning is performed on both real and synthetic data simulating temporal and visual attacks undergone by videos for our different tasks
This solution for matching and detecting copied videos has been developed by AImageLab and Facebook AI Research and is now being used in production scale at Facebook to detect harmful content.
See the official announcement on the Facebook newsroom website.
Paper
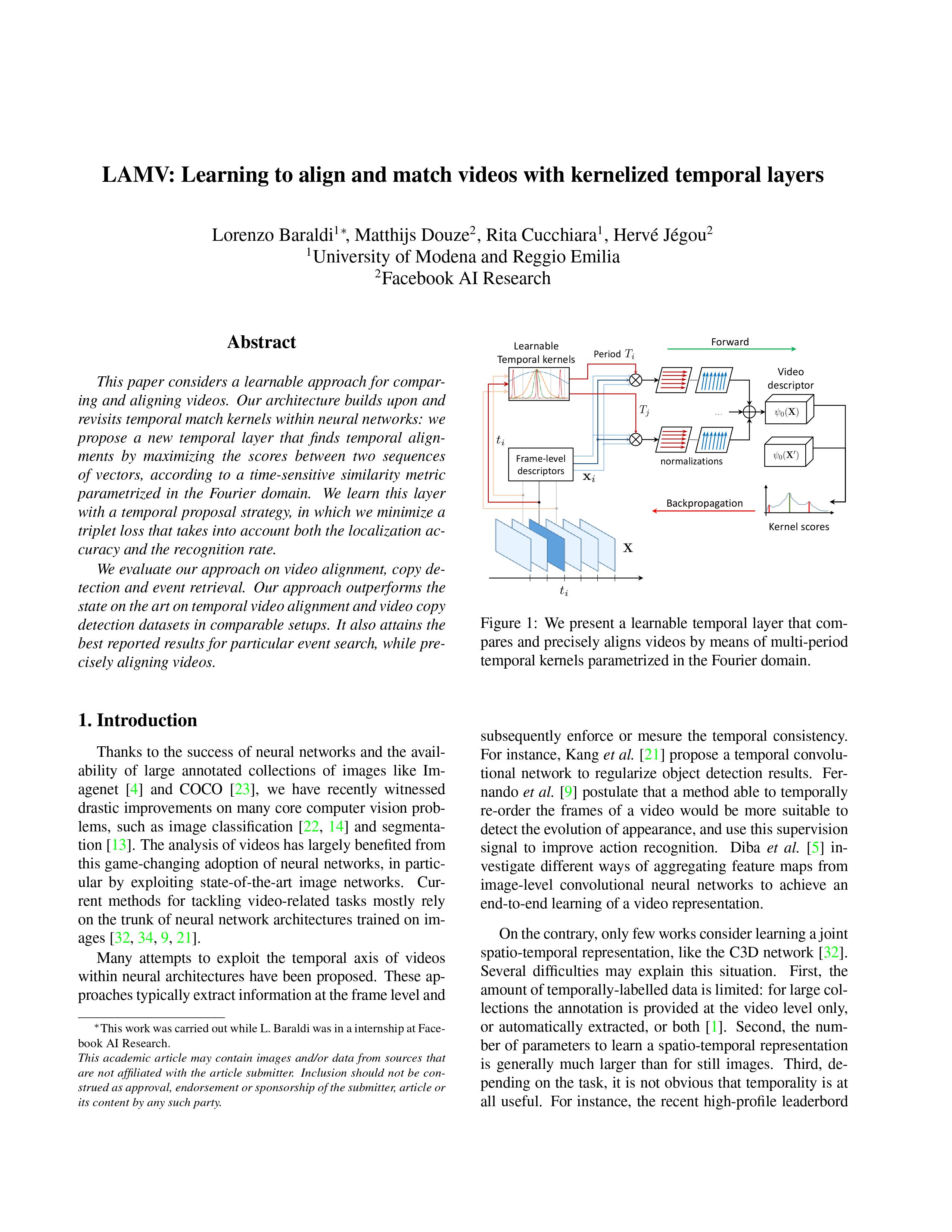
LAMV: Learning to align and match videos with kernelized temporal layers
L. Baraldi, M. Douze, R. Cucchiara, H. Jegou
CVPR 2018
Publications
1 |
Baraldi, Lorenzo; Douze, Matthijs; Cucchiara, Rita; Jégou, Hervé
"LAMV: Learning to align and match videos with kernelized temporal layers"
2018 IEEE/CVF Conference on Computer Vision and Pattern Recognition,
Salt Lake City, UT, USA, USA,
pp. 7804
-7813
,
June 18-22,
2018
| DOI: 10.1109/CVPR.2018.00814
Conference
![]() |