
Learning to Generate Faces from RGB and Depth data
We investigate the Face Generation task, inspired by the Privileged Information approach, in which the main idea is to add knowledge at training time -- the generated faces -- in order to improve the performance of the presented systems at testing time.
Our main research questions are:
- Is it possible to generate gray-level face images from the corresponding depth ones?
- Is it possible to generate depth face maps from the corresponding gray-level ones?
Experimental results confirm the effectiveness of this research investigation.
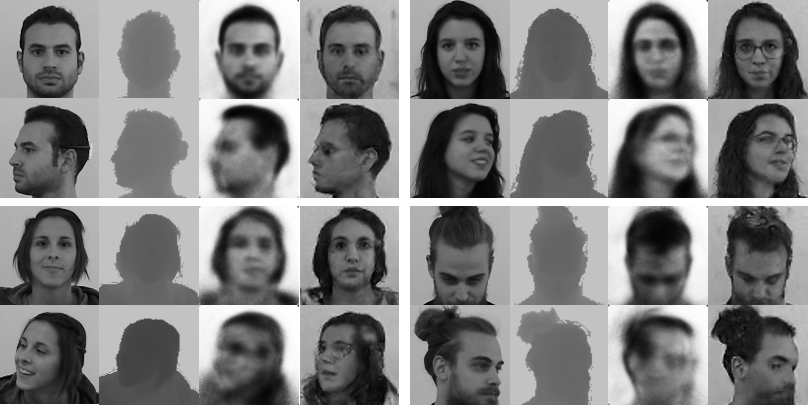
From Depth to RGB
In CVPR 2017 we proposed the first version of the Face-from-Depth model, based on an autoencoder-like architecture.
You can find further details here.
A new Face-from-Depth architecture, which exploits the recent Deterministic Conditional GAN models to reconstruct gray-level face images has been submitted at TPAMI.
To the best of our knowledge, this is one of the first proposals to generate intensity images from depth data for the head pose estimation task with an adversarial approach. Moreover, we evaluate and check the overall quality of the computed face images and results confirm their high quality and accuracy.
You can find further details here.
Pandora dataset (CVPR 2017)
Face-from-Depth v1 (CVPR 2017)
Face-from-Depth v2 (PAMI 2018)
PAMI 2018 paper
From RGB to Depth
By following an image-to-image approach, we combine the advantages of supervised learning and adversarial training, proposing a conditional Generative Adversarial Network that effectively learns to translate intensity face images into the corresponding depth maps.
Furthermore, we show that the model is capable of predicting distinctive facial details by testing the generated depth maps through a deep model trained on authentic depth maps for the face verification task.
Considerations:
- The detail accuracy of the proposed model is quite good, compared with the tested competitors;
- δ-metrics commonly used (δ<1.25, δ<1.25^2, δ<1.25^3), are effective to check the overall quality of depth maps generated from landscapes or wide-angle scenes, but the threshold value is too high to take fine details into account;
- We introduce a new set of δ-metrics (δ<1.25^(1/2), δ<1.25^(1/3), δ<1.25^(1/4)) with harder thresholds;
Detail accuracy is still an open problem with Conditional GANs and Depth Estimation!
Finally, we note that our approach is able to produce overall accurate views of the generated facial depth maps, preserving the shape of the face and the garments.
3DV 2018 paper
Publications
1 |
Borghi, Guido; Fabbri, Matteo; Vezzani, Roberto; Calderara, Simone; Cucchiara, Rita
"Face-from-Depth for Head Pose Estimation on Depth Images"
IEEE TRANSACTIONS ON PATTERN ANALYSIS AND MACHINE INTELLIGENCE,
vol. 42,
pp. 596
-609
,
2020
| DOI: 10.1109/TPAMI.2018.2885472
Journal
![]() |
2 |
Pini, Stefano; Grazioli, Filippo; Borghi, Guido; Vezzani, Roberto; Cucchiara, Rita
"Learning to Generate Facial Depth Maps"
Proceedings of the 6th international conference on 3D Vision (3DV),
Verona,
5-8 September,
2018
| DOI: 10.1109/3DV.2018.00078
Conference
![]() |
3 |
Borghi, Guido; Venturelli, Marco; Vezzani, Roberto; Cucchiara, Rita
"POSEidon: Face-from-Depth for Driver Pose Estimation"
Proceedings of the IEEE Conference on Computer Vision and Pattern Recognition (CVPR),
vol. 2017-,
Honolulu, Hawaii,
pp. 5494
-5503
,
July, 22-25, 2017,
2017
| DOI: 10.1109/CVPR.2017.583
Conference
![]() |